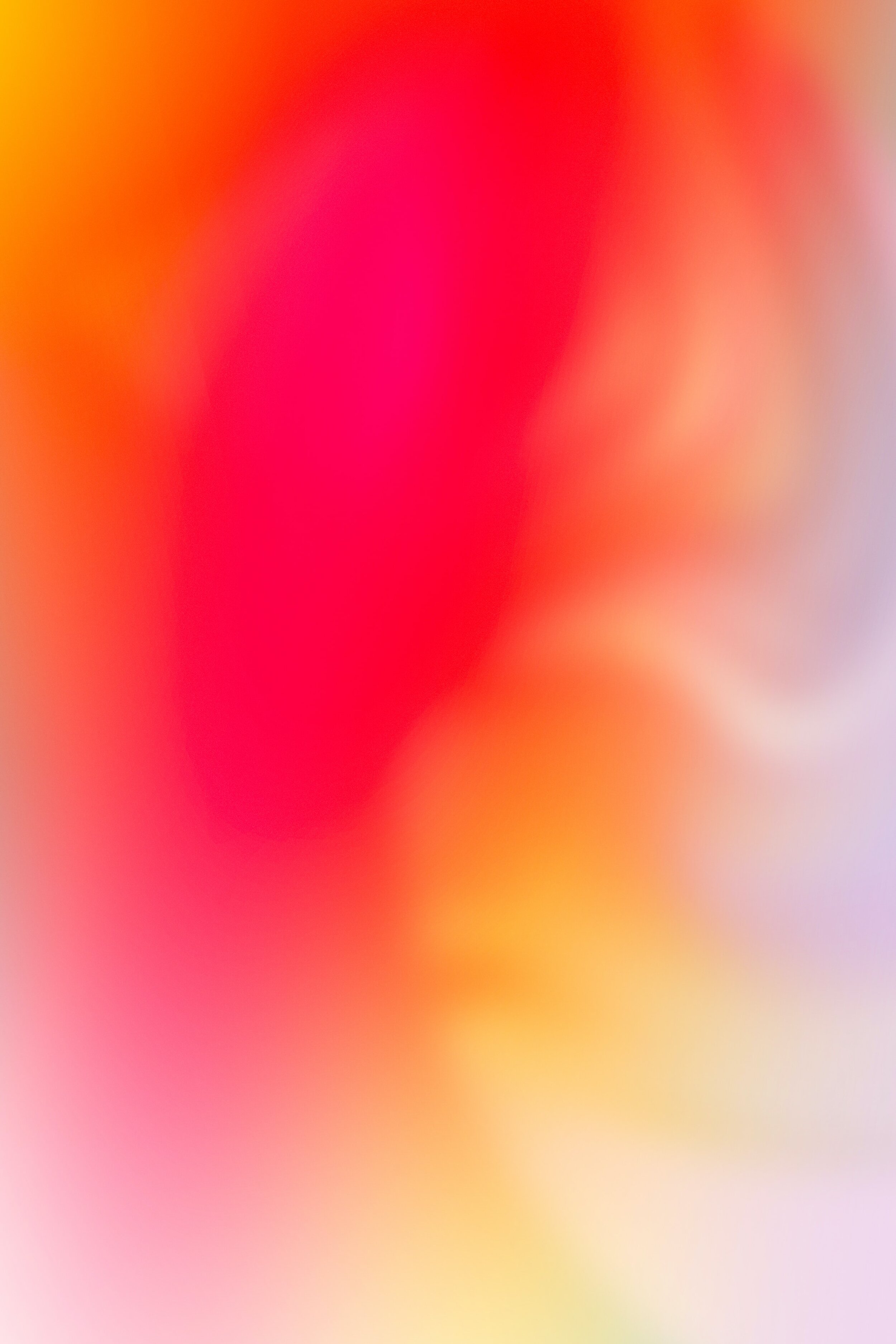
August 19 2024 • Episode 018
Aleksander Fabijan: Microsoft - The Experimentation Flywheel: Growing A/B Testing Momentum at Scale
“ Experimentation teams must focus on doing the basics right, performing simple, high-quality experiments often. This is the winning recipe for the experimentation flywheel to keep spinning faster and faster. Introducing too much complexity can make it difficult to detect learnings due to errors, or very difficult analyses ”.
Aleksander Fabijan is Senior Product Manager at Microsoft. He is part of a 60+ team of Data Scientists, Software Engineers and Program Managers that form the Experimentation Platform (ExP) team. The ExP team is responsible for one of the largest and most cutting-edge online experimentation systems in the industry. The mission of the team is to accelerate innovation at Microsoft through trustworthy experimentation. Major products such as Bing, MS Office, Skype, Xbox, Windows, and MS Teams all use the ExP platform to perform online controlled experiments.
Aleksander holds a PhD from Malmo University where he conducted his research at Software Centre, a European collaboration of companies and universities accelerating the adoption of novel software engineering approaches. He has published and co-authored many influential research papers on large-scale online controlled experimentation. Key research interests - running trustworthy experiments, experimentation system architecture, and examination of experiments (analysis).
His passions include data-driven decision-making using A/B testing, AI system design, API design, User Experience (UX) and large-scale experimentation.
* The views expressed in this podcast are Alek’s personal perspective and not necessarily representative of Microsoft.
Get the transcript
Episode 018 - Aleksander Fabijan - Microsoft - The Experimentation Flywheel: Growing A/B Testing Momentum at Scale
Gavin Bryant 0:03
Hello and welcome to the Experimentation Masters Podcast. Today I would like to welcome Aleksander Fabijan to the show. Aleksander is currently Senior Product Manager at Microsoft, where he works as part of the experimentation platform team, one of the largest and most cutting-edge online experimentation systems in the industry. Holding a PhD from Malmo University Sweden he has published and co-authored many influential research papers on large scale online controlled experimentation. Welcome to the show Aleksander.
Aleksander Fabijan 0:41
Great, thanks for having me. Hello, everyone.
Gavin Bryant 0:45
I've been meaning to reach out for some time to talk to you about the experimentation flywheel paper. Aleksander, I thank you so much for giving up some of your time and agreeing to have a chat about the paper. That will be largely the focus discussion on the podcast today. Before we get into discussing the paper proper, I wanted to learn a little bit more about you and your journey you have a very interesting journey and background crossing Slovenia through Europe and to the US. Could you give us a little bit of background on your journey today please?
Aleksander Fabijan 1:07
That's awesome.
Aleksander Fabijan 1:30
Happy to share. As you've mentioned in the beginning, I work now with Microsoft's experimentation platform team. And I've been here for over six years. And about half of that I've been working as a data scientist, and the other half was a product manager. And both halves have been very fun and very inspirational for me. As you've probably seen in, you know, the papers and the blogs that I'd been co-authoring or publishing directly. A/B testing, and the broader topic of online controlled experimentation is, you know, a great passion of mine. But I didn't start my journey here, just kind of-- how you said it all started. Very early on, after I've graduated from computer science. That was still back in Slovenia.
I've met my wife, who was Austrian, and we immediately moved to Vienna, in Austria. And there I found a job where I worked as an engineer. And one part of my jobs was to understand the data that we were collecting for security purposes, for PCI compliance, I'm sure a lot of your audiences may be familiar of PCI compliance on online shopping. And as I was kind of doing that work, I've realized, oh, this data can be useful to maybe inform some other decisions as well. But this was maybe 15 years ago already. And the role of data driven development hasn't really been widespread back then. But I've seen this great opportunity to do a PhD in the area of data driven development in Sweden.
So that's what brought me to Sweden. And I've started to work with a lot of great companies up in the Nordics, who've been always using data for various purposes, and wanting to become more data driven. And I learned all about basic analytics all the way up to A/B testing or experimentation, as some of you might call it. That brought me to Microsoft EXP, as first as an intern many, many years ago, and then as a full-time data scientist, and I never looked back, I still think strongly that A/B testing is one of the greatest tools in the arsenal that we have as scientists, product managers or executives and yeah, that's what do you see reflected in many of those papers that you mentioned. I hope.
Gavin Bryant 4:22
One of the things that I wanted to explore with you a little bit further and dive a little bit deeper on was your PhD studies in Sweden. What was some of those early learnings that you took from Sweden to Microsoft around A/B testing?
Aleksander Fabijan 4:39
I think the learnings were both directions, as I've worked with companies in the Nordics, I've learned about some great practices of how they operate with telemetry and even with qualitative studies and how they put them all together to make sense of great product development. And those learnings were really the baseline that I could use at other places where using telemetry data was the default and there was less of the quality of side of it.
So, you know, it wasn't only one direction that there would be learnings going in one way, I think there was a lot of commonalities there that we could benefit from companies across the world. But if there's one thing that I observed in the Nordics is that the companies have been very eager to move fast and try new things which is very common also, I see the cup of with many companies here in the States, whereas I live previously more in German World and Slovenian World, there has been more friction in getting the change to be implemented.
So, moving fast that's important. But I was an intern at Microsoft, I also brought back I think some useful knowledge to the community that I was living in, the A/B testing, back in the days has not been really widely publicized, I think, across the industry. So, our team-- the EXP team has been, I think, driving a lot of those publications to Ronnie and to other folks on the team that like, this is a really impactful tool to make good decisions and product experiences. But how do we actually do it in practice, you know, it was still early days. So like, I was able to bring some of that back to the community that I live in Sweden. And I think to the broader community, because I've published quite a lot of that knowledge.
In fact, maybe some of your audience might be familiar with the maturity model, that was actually one of the first major publications in my work, where we described, what are some of the stages that companies or product teams go through, when they start running A/B tests and then scale all the way to hundreds or 1000s of A/B tests. And if you had a chance to look at that publication, in that page and it has been reposted or republished many, many times across different companies and organizations. You probably noticed that there's a few different dimensions to that path and that the story itself is not only technical, like a lot of the teams have maybe initially thought of this but it involves removing friction, changing culture, getting on top of measurement and understanding how to make tradeoffs and so on so yeah, I'll leave it there.
Gavin Bryant 8:10
For those that haven't read the maturity model, crawl, walk, run, and fly. So, I will post a link to that. That paper in the show notes for those who want to follow up on it. One of the things that just stuck out to me there, Aleksander was, you're suggesting that A/B testing is one of the greatest tools. One of the things that stuck out to me recently in the marketplace, like, late last year was from Duolingo, where within the shareholder letter and their investor memo, they dedicated half a page to experimentation. And there was specific reference there of the growth and success of the company and a direct attribution to a culture of A/B testing and the A/B testing program, which I thought was a real shift in the industry that companies are now going on the record publicly to investors in the market and communicating how experimentation is being utilized to develop and extend competitive advantage.
Aleksander Fabijan 9:24
Yeah, I think I've seen that article. And on one side, I'm really happy to see that both leadership executives and boards are aware of the great impact that A/B testing can have on product and making decisions. At the same time, I want to immediately caution here that-- At end of the day, this is a powerful tool. A powerful mechanism to make the right decisions. But you make those decisions against metrics.
So, in here, we have to be really careful that-- I hope that your audience, you know, is aware of this that we have, when we look at products, when we look at, you know, different solutions that are running A/B test today is that we don't optimize for short term gains or something that would like help us bring short term improvements, but we look at user experience and success on how users can actually have a better time with the product that we're building, and measure that and move that, right.
So, it's great to see that-- I don't know the specifics of that particular report, enough to what were they reporting on, but at least in my view, that the main point I want to raise here is that we need to always be cautious to have great metrics that measure user success on the long term, and use that as our A/B testing goals. And, yes, that's what actually is going to lead us to successful good experiences, returning users, and potentially can lead to that kind of results that you were also saying, so I don't know how long their period was, what they have done, but immediately this is important to call out here.
Gavin Bryant 11:30
Just holding that thought on metrics for the moment one of the things that I wanted to ask you working as a Senior Product Manager with experimentation day to day, across the business with different teams, what are some of the biggest struggles and challenges that your teams having with A/B Testing, day in, day out?
Aleksander Fabijan 11:54
So, on experimentation platform team, we have a really unique role, where we focus on making the platform great for our customers, which in this case, are internal product teams at Microsoft. So, my role is to enable those teams to have a great experience in using A/B testing platform, which means creating good UX experience, building SDKs, API, so they can integrate A/B testing into their workflows, if necessary. And just in general, it's all about enabling our teams to run A/B tests at scale.
So, I don't personally go and run a lot of those A/B tests on my own, but I can see the struggles across multiple teams of where they begin, and it typically all starts with motivation. And why would someone want to run even A/B tests, so a lot of that initial friction starts at that point. Because when you work in a very large company across many different products and divisions, you can probably imagine that there's not many pockets where this is a new thing, the A/B testing has had a great presence in online services, like the search engines and ranking. But there's other places where it hasn’t had such huge penetration yet.
So, it starts with why and for that we usually have some classes to educate with good examples and share great A/B tests and learnings from other teams. And we overcome that part. And then when it comes to actually running a specific A/B test, as teams have decided that they will do that, there's always a friction of how do you now set this up? So, it's gonna be running correctly, how do you integrate it on a code level correctly and that friction can be removed today with some great automation even the large language models can help in that cases but we need to make that path as easy as possible to write and that's my job as product manager as well like help the teams make the smooth. I feel going that A/B testing has maybe for many years been seen as a separate product to the product development that you may be-- I'm developing my product and writing my code and now, I have something done, I'm gonna go and set up my A/B tests in some tools somewhere in the run and I'll make some conclusions and ship. I think, in right now we're in a state at least with many enough teams have worked with and even other companies to collaborate with where this is becoming-- And must become a well-integrated part of the development flow.
So, to remove that friction that I was saying earlier, it's all about integrating your A/B testing, setup, analysis, alerts as they come up with results into your existing flow as opposed to having this extra flow. I'm not sure if that makes sense Gavin but I'll let you let you share thoughts on that later if you have them.
So that is the second friction. And then finally, the friction is on the analysis. People see results. People see metrics that have moved. Now it's time to make tradeoffs. It's very easy sometimes to blame the tool for not being intuitive or good enough to be able to make a decision. But at the end of the day, all returns back to, do you have the right metric that you're measuring? And do you know what's important, so you can make that trade off. So, trying to remove the friction from the tradeoffs and make that possible and easy, is its own problem initiative, as you can probably imagine, and product can help with that. But it must be a much more complete approach to get that one done.
Gavin Bryant 16:30
One of the things that I just wanted to touch on briefly there, you mentioned that the penetration with A/B testing, it varies from team-to-team function to function. And you suggested that a good way to increase penetration in teams where the uptake is maybe a little bit lower is firstly around education and highlighting the benefits. From my experience, I'm a big believer in that experimentation-- A/B testing seen to be believed. And once people experience it, there's no turning back. It is just such a superior way to learn, to develop product and to make decisions. Do you find that's the case, that once people take that initial step and perform those initial experiments, that they're converted quite quickly.
Aleksander Fabijan 17:25
I think that's the case. And that's the case, if the A/B test has been conducted in a semi good quality way. And by that, I mean, the person who then sees the results is amazed about them, also appreciate the time that they have put into it. And by that, I mean, they didn't have to spend unlimited amount of time building some data pipeline to get there. They didn't get a result that didn't have the metrics they were interested in. And or they didn't get help to understand why that metric has moved.
So, I think what you're saying there is if an A/B test is of quality, and no matter if the result is positive or negative, that's something that changes I think here a lot, oftentimes positive results do make more excitement. But even a negative result is oftentimes valuable to stop investing. I think the key there is that there has been a benefit for a long to medium investment for that person. And once they see that benefit, they will never go away from it, they will never go away from it. And I think that there's this common misconception that A/B testing can be used only as a target to improve something. Maybe we can talk about this more later. But I've seen two key scenarios where A/B testing can be adopted.
One of them is the one that is frequently discussed in our papers. And we pop in a lot of the marketing materials that I find in internet habit. Whereas I want to improve a metric retention of users and different ideas and test that's for sure one possible approach to start. But the approach that we've seen work great across the industry in safe velocity. Engineers, even product managers have to deliver certain features, certain ideas, certain new things just as a sake of improvements to the product sometimes, and they're not there yet to actually measure the improvements but they're always there when things go wrong.
So, I encourage your audience to look at our paper and safe velocity, which really talks about how making A/B testing just a default step in the release process, for every change that comes through, can really transform the culture within the team that is really, really instrumental on how to get that adoption that buy, if a team is maybe not ready does not understand the value and doesn't have the right metrics.
So just being able to measure what is going out holding out half of your population from this new thing. And just looking at your guardrails has any error rates went up as the performance by any chance decreased, and we didn't expect that, well, there's going to be cases where you're going to catch issues that you don't expect. And you're going to catch them and then you get your champions who are going to start to believe in this methodology and start to use it also for the scenarios of improving the product. So that's the same philosophy. Whenever there is no good adoption with; I want to optimize approach, sales velocity is always there, it builds up the momentum and you have the right infrastructure in place to then do your optimizations if you choose to do them.
Gavin Bryant 21:13
One of the things that really stuck out to me there was notionally the need to abstract away all the complexity to reduce the human cost of experimentation as much as possible. And to make that process is seemly, and as easy as possible to enable people to spin up their experiments at low overhead, and to your point that that will then you know, help them to perform more experiments. However, if there's too much friction, and then human cost is too high, then it will potentially drive them away. Okay, let's flip the script a little bit now. And let's focus our discussion around some specific questions on the flywheel paper.
So just before we dive into some questions from the paper, I just wanted to highlight for the audience who haven't seen the flywheel it has five fundamental steps to it.
Step one is, perform more tests.
Step two, quantify value.
Step three is, increase interest.
Step four is, invest in infrastructure and data quality.
And step five, is increase automation.
One of the first questions that I had in the paper and something that really stuck out to me was a little macro flywheel that you had in the paper, that the value investment flywheel, and in my experience, I've been really lucky and fortunate to establish and scale an experimentation program from scratch in a large organization. And what it felt like to me was, I was a startup founder in a large organization. For the first 12 months, it was clearly just lobbying and influencing to get that initial investment and funding to establish the function and the team. And then it felt like every six months, we were working through this process of value and investment that while we continued to demonstrate value, the business continued to invest and this process continued on for many, many years. So, I'm interested to understand your experience with the value investment flywheel.
Aleksander Fabijan 23:43
Yeah, at the end of the day, it's all about what we put in what do we get back of it, return on investment. So, the same goes with A/B testing, we touched on it earlier. I think when it comes to A/B testing, there's kind of three dimensions of value, at least from the angle of where I'm coming from, like, one is the value the teams that are running A/B tests are getting right and in here, this can be very easily quantified. And I gave an example earlier with sales velocity, if A/B testing is just a step in your release path, you're going to detect certain things, just the nature of the greatness of A/B testing being so sensitive.
And so, pinpointing to your changes. You don't want to take some things that are bad, some things that are hurting the product, count them, share them and say how fast turn them off. Because the end of the day, one of the greatest benefits that we've seen, as for our engineers, for the people we work with is when an A/B tests the texts, the regression or something that you were not expecting, turning it off is a single click, or you can even up the, you know, in our cases, we even automate those things. Not running an A/B test may require a deployment and going into the system again, which can take a lot of time.
So how much time have you saved here, just by clicking the button to turn something off, and how you've protected your half of your users from even seeing this in the first place. And make sure that this didn't go out, despite all the rigorous testing you've done internally. So, issues detected the time to mitigation in the safe velocity scenarios, incredible power that A/B testing brings there for that part of it.
And on the other side, if you've been running a maybe testing program for maybe a year or longer, at that point, you were probably really looking at the measurement dimension of the growth model and started to think about product wide guardrails and success metrics, potentially. And what are the gains that you've actually gotten to those metrics? Those things are quantifiable. You can look at carefully across multiple A/B tests, what were those gains, and tie it back to the changes that actually made them happen. But most importantly, the changes that didn't make those gains happen, and probably some executive sponsor has been sponsoring. And said, let's try this, I think this is gonna work but then you show through the A/B test this is not.
So those wins, the small wins that you get through those A/B tests, either in a positive or negative directions, they have to be captured, they have to be celebrated. And it's been really important, from my perspective on the team dimension of running A/B tests. But oftentimes, you're not gonna celebrate only that, because the people who are running A/B tests, they're not the only part of the flywheel, there's also the champions who are helping to set up this environment for A/B testing to happen. But like you, Gavin, that going in the organization and thinks about metrics, what should be measured, setting up those metrics, validating those metrics, and in making sure that when those metrics move, there is a path for those people to come and understand why they have moved.
So, as you're measuring the success and the value of the teams running A/B tests, you should also be measuring the success and the value that the enablers provide like yourself, when you go into team and here most of it is sad. But the most value usually comes when it's almost invisible, when teams can be self-served, they can open some tool that helps them understand right away why this metric has moved, that you have provided them insights on, then that's a great time to success the A/B test can be made a decision on very quickly. In contrast, if teams are stuck, they see something positive, something negative, but they don't have a path forward to understand what has happened and how to make a decision, then this layer is missing of support that helps them understand in the greater level of why this has moved in this way.
So those are the two dimensions, we have the teams running A/B tests and the teams that support those teams that run A/B tests, both of them provide value. And for both of them, we have to capture the value that those people create. And then at the end of the day, at least in my case, it also boils down to the platform team itself. Like I'm in experimentation platform EXP or A/B testing platform. So, platforms have a well-defined set of service layer agreements and measures that can be used to measure how well the platform is performing. So yeah, as you're doing your A/B testing program, there's multiple dimensions of value that needs to be measured and captured to really grasp the full benefit of A/B testing to your context.
Gavin Bryant 29:36
Yeah, I think that's a really good point that when teams are thinking about how to quantify value, particularly in those early days when it's very critical, important to potentially to think of those sort of three, the key groups there that, you know, the broader the broader value to business, the issues detection and then monitoring, and then thinking more broadly across the product and the impact to key metrics, but then also, as you mentioned, looking at contentious ideas where there's maybe a lot of conjecture, so stopping things as well, where there is no value or benefit to users.
So yeah, three or four key categories for teams to think about. One of the things that I wanted to dive in a little bit deeper on and you touched on this is-- From my experience, though, those early months and years of any experimentation program are really critical for survival. And they can really make or break a program, while that program, can continue to demonstrate value, it will continue to thrive. What are some of your tips and strategies to help teams to get runs on the board and establish those quick wins early on in program inception?
Aleksander Fabijan 31:05
So right in the beginning, as you're kind of like starting to-- So I think in those cases, there's really three things that are important that that that I found work as a success 100% of the time, okay, it's a secret recipe. The first one is you need to test a trifold I call it a Cerberus; you need to test a Cerberus idea. It's a trifold idea, it needs to have three things, it needs to be visible. So, this idea needs to be visible both to the leadership and also to your users.
So, this is the first part of this Cerberus idea needs to be visible. People need to care about that part of the product. And you get to some visitors and some users and leadership, maybe in your colleagues, they have some disagreements, some conversations about what should be done there. So, this I call a visible idea, there's a good audience out there that cares about an idea, okay. This idea also needs to be simple. Like, I've seen on the market, there's this A/B testing platform and multi variants. And they support all sorts of advanced statistical techniques that you know, as data scientists we like. But in practice, this is all very, very difficult to execute correctly.
So, this idea just has to be an A and B, it's okay it's a, b, and c, I'm okay with that. But it doesn't have to be a complex change that needs to fundamentally redesign architecture of your service or redesign your UX, it just needs to be an idea that you can simply make two versions of it either your ranker model that returns results, you have two of those that do them in different order, or you have a UX that slightly different versions of it. I think those are some great examples. But going into some something super complex, like a change where a whole infrastructure is being moved from one type of cloud service to a different type of cloud service and might involve a lot of months and to be working, it's gonna be very complex to set up. It's not a great idea to start with. So that one is not simple.
So, we have visible and simple. And the third thing is, you have telemetry for your idea. And this is your Cerberus idea. You have telemetry, so you get some Cerberus already what the system or user interactions are. It's simple to do an A & B version of this idea. And it's visible to leadership in your users, that's a great Cerberus idea. But that won't be enough. You also need to have a strong champion that's going to work with you. And beliefs an A/B testing, at least beliefs you that A/B testing is great. That goes and connects with the right people to make executing on this idea possible. It's no matter what I do in life, and what I've seen, happen-- People are everything. People are great. But also, every problem at the end of the day, it's a people problem.
So having a great champion is going to really make sure that your Cerberus idea can lead into a successful early win in a fast way because that champion will find it a shortcut to get it done quickly, and then get the right people engaged to promote it around the team. So, I think that's I hope going to be helpful.
Gavin Bryant 35:00
I think that's a great little heuristic, visible simple and you can execute. And one of the things that stuck out to me was; what was notionally around the type of testing that teams just need to get really skilled and capable of performing high quality A/B tests as the cornerstone of the program and that will be their, the bread and butter of the program year in and year out and not be too concerned with running off and trying to perform more advanced techniques before they're ready for it. Just do the basics right.
Aleksander Fabijan 35:42
Doing the basics rights, and doing them often is the winning recipe for that flywheel to keep spinning faster and faster. Introducing complexity is great your hackathons and the extra activities that you might want to do on top of it, you try out new things that kind of program. But if you're really trying to become successful in measuring the impact of your changes, do it in a simple way, compare A, B, C to each other. And you will see which one actually works and take those learnings forward and avoid designs of A/B tests that are complex to set up require a lot of engineering input and a lot of data science input to even get started.
And it will also require a lot of effort to be analyzed, in the end of the day, you might actually not learn a lot of useful things from those complex setups, because you won't be able to tie them to specific change that you've done, maybe because of lack of power, because of something else that will go wrong. And just to kind of say this, Gavin, there's probably been a few 100,000 A/B tests that I've had a chance to see, work with, analyze or operate in some way. And there is enough complexity, just with A/B tests, that things can go wrong. We can talk about SRM, so that things, you know, if you have time for it, and you've probably read a lot of our publications on these topics, but this is difficult enough. And this is often novel enough for people to take on as a new activity in the company. So don't make it harder for them, keep it simple, and make it valuable.
Gavin Bryant 37:49
Okay, let's quickly talk about that. What are some of the things that can go wrong?
Aleksander Fabijan 37:56
Well in an A/B test, you essentially can get both the design and execution wrong, those are the two major parts. And by design, I mean, here, you maybe don't have a stable unit that you're randomizing on and maybe you're not including enough instances, enough users, maybe devices in depending what different companies use, that will actually lead to not enough power.
And then in execution side of it, it all boils down to the telemetry that you're looking at, how the data is being hooked together and joined and combined into a meaningful metric. And there is a ton of things that can go wrong, data pipeline, at some point, we wrote a paper on a problem called sample ratio mismatch, which I'm sure your audience is probably, maybe already familiar with, I hope they are. This is one of the most common problems. But let me rephrase that sample ratio mismatch is our ultimate detector of the problems that we can have in A/B tests.
So, while there can be 100 different things that could go wrong with an A/B test, the sample ratio mismatch is the tool that helps us detects that one of those 100 different things have gone wrong. So, the taxonomy on sample ratio mismatch causes then explains what are some of the more possible things that could have gone wrong and which ones are less prominent typically, doesn't get it in your situation. But the learning here is, things will go wrong in A/B tests, and you need to have the right guardrails to detect when they go wrong. The sample ratio mismatch is one such guardrail and every single day, I keep seeing an A/B test with a sampler ratio mismatch in some way or another, because of data, and most of the time, it's because of some telemetry that has been impacted by the variant in some way, because of a condition on how the data is being looked at. It is not fair across those variants, or some other other issue.
So, Gavin, make sure you test, your A/B-- I'm hoping you're already testing your A/B test with the first sample ratio mismatch and following the taxonomy to figure out why you're having it.
Gavin Bryant 40:40
Yep, one of the things you touched on initially Aleksander was power. Do you think that underpowered experiments is probably one of the most common challenges that teams face particularly when there's lower traffic sites?
Aleksander Fabijan 40:58
Yeah, underpowered A/B tests are actually quite dangerous, because you might get the wrong conclusion there. Nowadays, you see some movement, or you don't see a movement. And the important part here is just to be cognizant about it, right? Well, I don't have enough, enough devices here to understand the movement and the sensitivity, I have to ask for something different. But what? The best thing here is that there is a lot of things that you can do differently to gain sensitivity. That's the universal power is gained, the sensitivity.
And it all starts with your design, we talked earlier, sometimes you just need to reduce the number of variants, let's test at least does it even matter, let's just test two variants instead of the four that you're distributing users into fewer variants, but that's really not the end solution, right? Then you have the statistical techniques like variance reduction, you can look at the paper from Alex Dang. Basically, you can use the data from the history to understand how your metrics react, and then as you combine that with the A/B test data, you can have your metrics much more sensitive that way. But it doesn't stop there either, right? Metrics themselves, they will be impacted by outliers.
So, you need to look at the data, do they have outliers, and make sure you're capping your averages correctly. But that is also not the solution. Sometimes, if you really have low power, you might just have to look at the indicator’s metrics, like percentage metrics, percent of devices, in some experience, because indicator metrics, like, they will be much more sensitive, and they won't be impacted by outliers.
So, there's a number of different things that you can do, where you have no power, you just have to be aware that you do have low power and be educated that this is an important concept. So, as we give an opportunity to people to create A/B test, we always ask them, would you like to give a hypothesis of which metric are you trying to impact and in the platform itself, we compute the power as well. And as we make sure that experiment, and the person is then informed that they had enough power to make a decision with that message, but maybe not enough power with the with the average metric that wasn't kept.
Gavin Bryant 43:49
Yeah, good point. Awareness is key. Number one.
Aleksander Fabijan 43:54
Yeah, but people always need to have a solution. Right? If you show them there's a problem without the next step, you're creating that friction that we talked about earlier. So, awareness is one and then there needs to be a path forward which is that champion and labor team that is providing in organizations that we talked to earlier.
Gavin Bryant 44:16
Let's talk about the flywheel specifically with regard to neglect. What are some of the elements of the flywheel that you observe teams neglect the most or neglect most commonly?
Aleksander Fabijan 44:37
I get invited to talk at different companies and different conferences. So, I have this joy to meet a lot of people across the industry that run A/B tests. And one thing that I've observed fairly commonly is that lowering the human cost of the A/B testing is the step which does not get enough attention. So, this is problematic, right? Because you can measure the value Oh, great, this was so impactful, everybody celebrating it.
So, everyone is interested in it. And that might lead to even improving the platform, builds some new features, teams are excited, they build new features in the platform. But then the next day A/B test has the same kind of effort to execute, it becomes less of a novelty and excitement for teams running A/B tests. And moreover, oh, this is a cost that tax we have to pay. And this is all great, we get this out. But it's expensive.
So, this is a very important step. And I mentioned a bit earlier that one key ingredient here is to integrate the A/B testing into your developer flow. So, it's seamless, right? So, it's part of it. And by that, to give some examples, it can mean that as setting up your variants, you get your chance to very easily in the code, define your AB tests without going into some external tool, right, necessarily, you can automate that part, or as you're getting the results, you can always view them in our case, for example, in our experimentation platform portal, but why not post them in the common channel that the whole team is talking about automatically, and you can just start talking about them there where team is already present, right.
So, make it super easy to get the results, your alerts, your findings, your code setup, just be part of your life cycle. And some of it you can read in our paper on A/B integrations, which we released last year. It's really been, I think, a step forward in reducing that friction in actually making it easier for people to have A/B tests and then.
Gavin Bryant 47:09
I loved your reference. Sorry, I loved your reference to the experimentation tax that no one likes taxes, we all want to pay lower taxes. So, yeah, a really good way of thinking about it simply is we want to reduce the amount of experimentation tax, our stakeholders, and users are paying. It's yeah, it's a really good little. Yeah, my analogy mental model. One of the things
Aleksander Fabijan 47:37
Analogy. I lived in Sweden, where this topic of taxes is fairly celebrated, and they're fairly high and in the United States, it's different, taxes in the Nordic countries are much higher.
Gavin Bryant 47:57
And, yeah, and I think, you know, tax in the US it varies by state too correct.
Aleksander Fabijan 48:05
Yes, certain states have a state tax, others don't. And it's a very complex setup that I have yet to master even after living many years here.
Gavin Bryant 48:23
One of the things that you've constantly referenced so far today, Aleksander is champions. And I interpret that two ways. So, champions, being senior leaders and executive leaders to support and endorse a program. But my second thought there is the champions on the ground every day who are embedded within the teams and our business units. The thread that keeps coming through is that these champions are critical to success on a day to day, week to week basis of the program. Could you elaborate on that a little bit more, please?
Aleksander Fabijan 49:04
Oh, yes, I can share my thoughts on this. So, like any initiative in A/B testing is no different than any other initiatives. It requires executive sponsorship and the ground support that you can share it. Here, I think it's also one other layer that I think you missed, let me try to explain. So, it all starts with that executive, as you said, that is willing to invest in A/B testing or any other initiative that there needs to be at least one in your organization that you can work closely with that kind of helps you sponsor this program.
That's a very important person to always inform about all your findings, value and the things we talked earlier that A/B testing program brings. And you mentioned that other side of the equation which is the champion who helps individual teams running A/B tests with their questions, with the metric design and just doing that hands on education and building new champions that can do that, and making that whole system grow, that's the other side of the equation. But then there is this middle tier champion, I think I like to call the liason which looks across a few different teams, the problems those teams are facing, and thinks about it, almost like a product manager does about the product roadmap, thinks it from a strategic roadmap for the initiative, as in what are some toolings or educational investments that we're going to need to do over the next quarter two or next year, that are gonna make the life of this teams running A/B test and the champions supporting them better to remove that friction of, of lowering the cost, right.
So, this is the one I think I didn't hear captured in your presentation there. You know, you have your executive sponsor, you have your liason and then you have the ground support, that type of a champion. And all three here, equally important, they just have a different responsibility and accountability. The support person is making sure that the day-to-day things are being unblocked.
The strategic liason was looking at two link that needs to be built or experiences improved, communicating the cross platform and other teams to see if they have experienced the same issues and bringing that up to the executive sponsor and platform sponsor, whatever whoever your stakeholder is, to find a sponsor and to continue to work on. I think that's the three most important types of champions that you need to have around.
Gavin Bryant 52:02
Great summary. Thank you for the clarification. One thing that I wanted to discuss with you just to close out our discussion on the flywheel. So, the flywheel paper was published three or four years ago now and I'm interested to understand how has your thinking and perspective evolved since initially publishing the paper.
Aleksander Fabijan 52:32
I've come to realize that the flywheels are the most important tools that we have to both show progress, but also to stay motivated and, and just create that energy. I mean, that in a, you know, on, on a level of developing an A/B testing program, especially when you see the new team struggling saying, we're not there, we cannot do this, you imprint them that and you show them there is a path, it's been really useful to have that tool, even for myself, to actually hit my goal and communicate, both on executive leadership sessions as well as you know, down to engineering sessions.
So, I maintain the opinion, the flywheels not only the A/B testing one, but just the general tool of the flywheel is really impactful, and simple tool that in an arsenal of product manager, leader or anyone that that is interested in growing some initiative and making something successful. That's one. And two, specifically with A/B testing flywheel. Small little flywheel have started to appear for each of those steps that you see them five steps and I've come to -- I work with many different teams.
So, I've gathered what's hard and I realized, okay, I need to make something simpler. For example, you know, that lowering the human cost part. We talked earlier about how do we make decisions quicker for a team right? Well, they need to understand why a metric has moved. So, let's build a small tool that does just that integrated into the platform measure and able to not do it quicker and then keep adding to that tool to make this small flywheel faster.
So, I think I hoping that audiences when they read the, the flywheel paper, they find inspiration but they don't pick it one to one into the organization. You have to adjust it a little bit I think, that's number one. You have to adjust it for your context. And two, as you're using it for a while, I think you're going to start seeing the small little flavors appearing across each of the steps and how you can make those steps also a little bit more iterative.
Gavin Bryant 55:09
Yeah, that's really, really good message and learning that the flywheels have been popularized, especially in growth circles around acquisition and retention and engagement, monetization. We always have macro flywheels. And the five steps of the macro flywheel is our universal flywheel, but to your point, to also have teams to think about what are the sub flywheels and the micro flywheels that exist under these larger macro flywheel. So, I think a really good practical message for teams to consider so that they can get that macro flywheel to spin faster. Okay, let's close out with our fast four closing questions.
What are you obsessing about right now can be work related can be personal?
Aleksander Fabijan 56:10
Oh, it's personal, it's NBA Western Conference Finals. My team Dellas Mavs, they are playing in a few moments actually, and they are in the finals of the Western Conference here. So, just crossing my fingers, they can win the best of four games against Timberwolves and get in the final of the NBA.
Gavin Bryant 56:36
Okay, good luck Mavs, fingers crossed.
Number two, thinking about one of your frustrations with A/B testing, do you have one?
Aleksander Fabijan 56:51
The A/B testing never frustrates me, the only frustration that I get is when there hasn't been done A/B testing, someone has just decided to ship a feature without safeguarding it first. Or if A/B testing is done in a very haphazard, low-quality way. So, if there's not the right quality checks, like sample ratio mismatch, if they're not applied, like, I cannot trust A/B test results. So, the reason people do A/B testing is because you want a quality decision. And if you avoid all the things that make it quality, then that frustrates me.
Gavin Bryant 57:38
Okay, number three, the biggest misconception about A/B testing.
Aleksander Fabijan 57:47
There's many, and one of the things that I came to realize is that A/B testing is also for A/B testing. So, we run A/B tests on our own A/B testing platform. And I can be as wrong as any other decision maker in any other product, I need to A/B test my ideas to, even if I work on A/B testing platform. So yeah, we published some of the-- Like, you can look at that example in the EXP blog on A/B infrastructure changes that are directly on our platform. And I encourage all the teams out there, that are thinking about offering or helping teams run A/B tests, you should run them too.
Gavin Bryant 58:38
Fantastic point to close. Now thinking about some resources that you'd recommend to our audience, so we will reference the maturity model, the flywheel paper. There's the Microsoft EXP blog, which has a lot of useful information on it. Ronnie has his online controlled experiments book. Is there any other resources that you'd like to highlight to our audience?
Aleksander Fabijan 59:08
I think one book that-- Some of you might be familiar with is the "Lean Startup" by Eric Ries, which just kind of describes how you, you want to be also iterative in creating your products and very early measure the value of the decisions you're putting in there. And I like that book a lot. I recommend it to audiences that are building out products because I think it naturally ties into A/B testing as the tool that you can use in today's world to make that effective, right. You cannot talk to all your users but you can definitely talk to them through A/B tests.
So, that's one book. And one book that podcasts and videos to that I really like is Extreme Ownership by Jocko Willinck. And I feel that people around us should really get to see this person Joshua present he's an ex-navy seal. And he talks about the importance of owning what you're doing and really being fully into it and executing and owning every good and bad decision that is out there. And again, this book has nothing to do with A/B testing, but you're not owning your product and decisions that you're doing. If you're not A/B testing in when you're building up software products, you're letting things to chance.
So, I think I've got extreme hunger will always look into making the right thing, the best thing to happen for your product and this book guides you why this is important and how it helps you with your growth in your leadership skills.
Gavin Bryant 1:00:57
Yeah, it's very good book. I have that one. It's a great recommendation. Now let's if people want to get in contact with you or have any questions, you're on LinkedIn is anywhere else people can find you.
Aleksander Fabijan 1:01:12
LinkedIn is the best place to find me, I have been so far very diligent in replying to folks that have reached out, I receive requests about research together and various things and I've so far always been trying to help out. So, feel free to reach out there.
Gavin Bryant 1:01:33
Thanks for the chat today. Aleksander really enjoyed it. And good luck to the Mavs today.
Aleksander Fabijan 1:01:39
Thank you. Thank you. I hope they win.
“ Sample Ratio Mismatch is our ultimate detector of the problems that we can have in A/B tests. When things go wrong, you need to have the right guardrails to detect when they go wrong. Every single day I see A/B tests with Sample Ratio Mismatch, due to data that is impacted in some way by the new variant ”.
Highlights
A/B Testing is one of the greatest tools in the arsenal that we have as Scientists, Product Managers and Executives. It is a powerful mechanism to make the right decisions - those decisions must be made against metrics
Moving fast is important - when studying his PhD in Sweden, Aleks observed Nordic companies being very eager to move fast and try new ideas
We shouldn’t be looking to optimise for short-term gains, and short-term improvements, rather looking at user experience more holistically to understand how users can have success with the product that you’re building
Struggles with experimentation start with motivation. Why would teams want to start performing A/B tests when there is a lot of friction? Initially, to reduce friction, educate sceptics by highlighting the benefits of A/B tests and providing practical examples and learning from other teams
Experimentation must become a well-integrated part of the development flow and release process. A/B testing setup, analysis and alerts must be integrated into existing workflows as opposed to having seperate workflows and processes. This is the only way to really transform culture within a team/organisation
All experiments need to ensure that they’re measuring the right metrics. Movements in metrics is the only way that you can evaluate trade-offs
Quantifying experimentation program value with the Value - Investment Flywheel (1). PROGRAM: meta analysis can be conducted to evaluate the impacts of A/B tests on the growth model. (2). PLATFORM: SLA’s to monitor and measure how well the experimentation platform is performing (3). PRODUCT: Teams are able to quantify the impact of their work (Positive/Negative) on user experience during the release path and (4). TEAM: Increase decision velocity and product velocity through self-service - teams are able to clearly understand impacts to key metrics and the path forward
The Secret Recipe to ensure a 100% success rate for experimentation program Quick Wins - VISIBILITY: choose an opportunity that is highly visible within the organisation and people care about - strongly held beliefs, strong convictions or disagreement. SIMPLICITY: the opportunity can be tested with a low-complexity A/B test (NOT a re-design of Architecture or UX) MEASUREMENT: you have a set of metrics to easily evaluate the test
Experimentation teams must focus on doing the basics right, performing simple, high-quality experiments often. This is the winning recipe for the experimentation flywheel to keep spinning faster and faster.
Sample Ratio Mismatch is our ultimate detector of the problems that we can have with A/B tests. When things go wrong, you need to have the right guardrails to detect when they go wrong
The most commonly neglected step of the flywheel is reducing the human cost of experimentation
Three Levels of Experimentation Champions - LEVEL 1: Executive Sponsorship to provide advocacy, provide investment and remove blockers LEVEL 2: Strategic Liaison to communicate between Ground Support, Executive Sponsors and Platform Sponsors on cross-platform experience issues and opportunities and LEVEL 3: Ground Support provide day-to-day, hands on support to teams at the coalface of experimentation to support experiment design, execution and troubleshooting
The Experimentation Flywheel exists at MACRO and MICRO level. The Macro level represents the 5 key steps of the Experimentation Flywheel: 1. Perform more experiments 2. Quantify value 3. Increase interest 4. Invest in infrastructure and data quality and 5. Automate. Think about which Micro Flywheels exist for each of the five Macro steps to increase Experimentation Flywheel velocity. Also, think about which Macro flywheels are connected (Increase interest < - > Perform more experiments)
In this episode we discuss:
An overview of the Microsoft Experimentation Team
Experimentation is a powerful tool to make the right decisions
Day-to-day struggles with experimentation at Microsoft
Increasing experimentation penetration in large organisations
Embedding experimentation as a default step in the release process
The Value-Investment Flywheel: Quantifying experimentation program value
Three steps to get early wins on the board
Sample Ratio Mismatch is the ultimate detector of problems
Areas of the experimentation flywheel that are most neglected
Three types of experimentation champions for program success
Flywheels are the most important tools to show progress
Success starts now.
Beat The Odds newsletter is jam packed with 100% practical lessons, strategies, and tips from world-leading experts in Experimentation, Innovation and Product Design.
So, join the hundreds of people who Beat The Odds every month with our help.