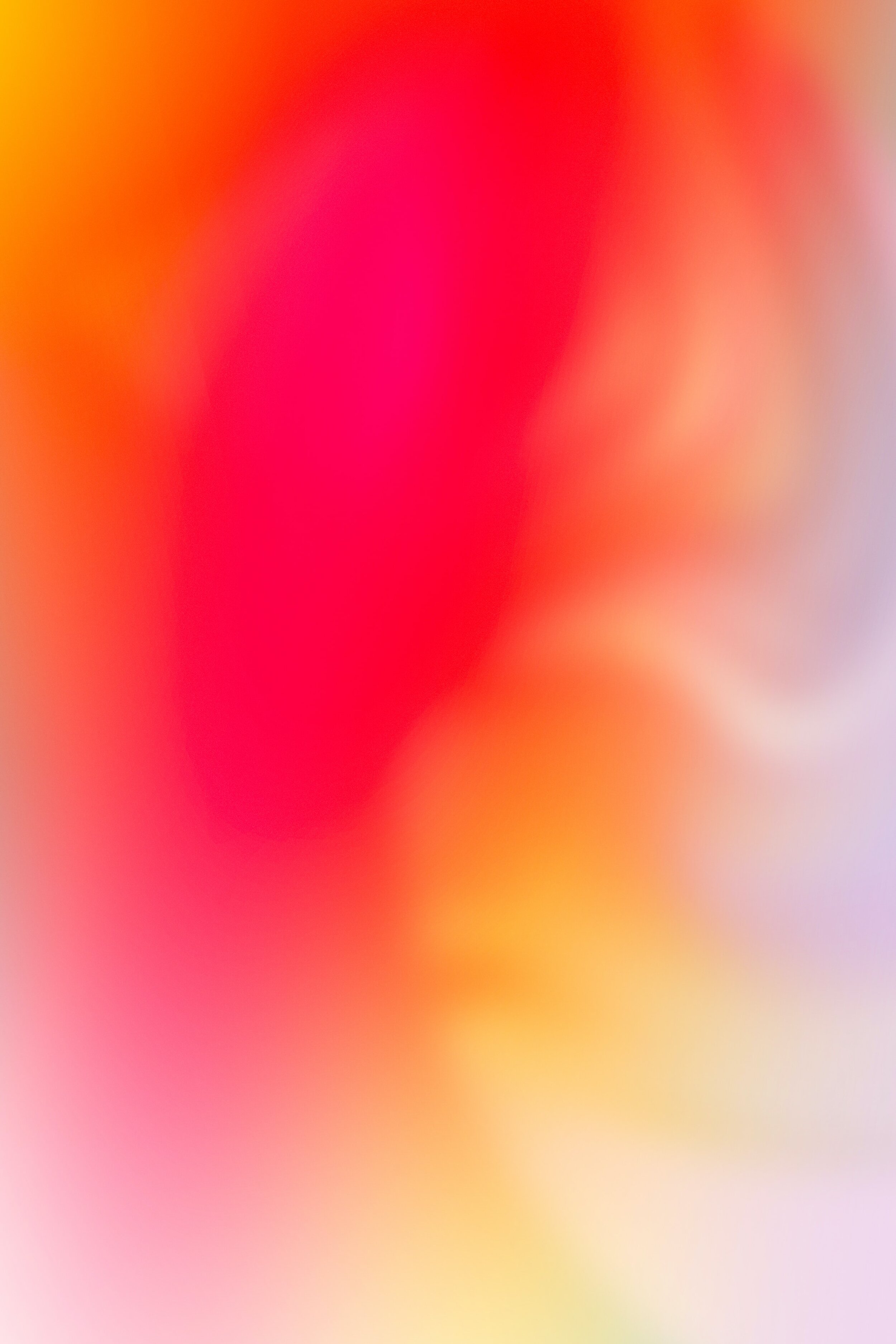
November 9 2024 • Episode 019
Jonas Alves - ABsmartly - Building & Scaling A World-Class Experimentation Platform
“ We built monitoring for interaction effects because people are afraid of them. Experiment interactions almost never happen. We have only had two alerts and one of them was a false positive. Interactions are so rare that people shouldn’t be concerned about it. People are conscious about what they are testing. They know when two things should not be tested at the same time. Interactions can happen when they are intentional and that's fine. You can start two different experiments with the intent that they will interact with each other, as long as you have a way to analyse them together ”
Jonas Alves is the CEO and co-founder of ABsmartly, a leading experimentation platform designed for trustworthy A/B testing. He has 16+ years-experience in the field of online experimentation working in-house and consulting, with experience including design and build of experimentation platforms, democratisation and scaling of experimentation programs and conducting training with experimentation teams.
Prior to ABsmartly, Jonas worked as an experimentation consultant, helping other organisations on their experimentation journey, including Catawiki.com, GetYourGuide, Viagogo, Adidas, Picnic.nl, MinDoktor.se, Match.com (OkCupid, Tinder), Remitly, SpotAHome, ParkMobile and many others.
He started with experimentation in 2008 at Booking.com. Here he democratised experimentation across the organisation to many development teams. By 2012, hundreds of people were performing experiments every day. As Product Owner (Experimentation), Jonas was responsible for experimentation training, new features, reporting and metrics.
When leaving Booking.com in 2015, the business was running more than 1000 experiments simultaneously and starting 100+ new experiments every single day.
Get the transcript
Episode 022 - Jonas Alves - ABsmartly - Building An Experimentation Platform
Gavin Bryant 00:06
Hello and welcome to the Experimentation Masters Podcast. Today, I would like to welcome Jonas Alves to the show. Jonas is currently the CEO and co-founder of AB smartly, a leading experimentation platform for trustworthy AB testing. He has 16 plus years’ experience in the experimentation industry, working in house and as a consultant, designing and building experimentation platforms, scaling experimentation programs and training experimentation teams. Jonas has worked for seven plus years @booking.com as head of experimentation, democratizing and scaling experimentation across the organization to perform 1000s of concurrent experiments by hundreds of people. Welcome to the show.
Jonas Alves 00:57
Thank you, Gavin. It's great to be here.
Gavin Bryant 01:00
And just a little brief intro for our audience that as many of you know that I'm running the 2024 Asia Pacific Experimentation Summit this year, and Jonas and team at AB smartly, are our proud partner for the event So expressing a huge amount of thanks and gratitude to the team at ABsmartly for helping and support a new event in the Asia Pacific region. So thank you kindly.
Jonas Alves 01:34
Thanks Gavin. It's a pleasure to help with that, and we are very interested in growing experimentation across the globe. So that area is very important for us as well.
Gavin Bryant 01:44
Okay, let's shift the focus back to you, and if you could just provide a little bit of an overview of your personal experimentation journey and background.
Jonas Alves 01:53
Sure, yeah. So basically, my journey with experimentation started when I joined booking.com in 2008. Booking was already experimenting at that time. They were running experiments since 2005 but it was a much more basic platform than what we ended up building later of course. Like in the beginning, it was very simple. We could see the conversion rate per variant, and we had a chi-square test for significance. And that was it like very basic but enough to run quite a few tests. And it was actually during that time that we ran some of the biggest successes in experimentation booking. I joined as a full-stack developer, and one of my first tasks was actually to improve the experiment tool and start running experiments in the website. So in the beginning, it was just myself and the designer running experiments. So a team of two very small, but smaller than what people know about booking now. And I made the tool a lot better. So I made it easier to track experiments to add metrics, because we could only see the conversion rate and we wanted to measure more stuff. So with one line of code, we could add track cancelation, track newsletter subscription, clicking a button. At some point, we had like 5000 metrics being tracked across the website. I became at some point, the head of experimentation networking, so responsible, not only for the tooling parts for the platform, but also for training, for the statistics, for new metrics being added. Pretty much everything related with the experimentation was falling on my lap. In around, I think 2012 I reached out to Ronny Kohavi at Microsoft, and he referred me to a colleague of him that worked with him at Microsoft, first at Amazon and later at Microsoft in the experimentation platform there as well. And that person that I referred to helped us a lot with the statistics of the tool as well. And then in 2015 when I decided to leave the company, booking was a factory like we were putting in production between 100 to 200 experiments every single day, and always having more than 1000 experiments running simultaneously across the website.
So really crazy, like, if you love experimentation that was like the best place to be. And then I passed the reins to Lukas Vermeer. That continued with my role at booking. I left to join--- I started consulting first. The idea was to join Marcio and Mario that are my co-founders now at ABsmartly, but they had joined the startup in Amsterdam, and basically they joined as co-founders. And I thought, well, that's something I would like to try as well. So I joined that company too, and we also built an experimentation platform for trip and year that company, but I started consulting before that, I went to Catawiki, and I worked one year as a product owner of experimentation Catawiki, they are now our customers. So first I helped them build an experimentation platform internally, and then they migrated. A few years later, they migrated to ABsmartly because everything that they wanted to build on top, we had done it already. So I helped many companies with experimentation as a consultant, and I got so many requests from those companies to help them build an experimentation platform internally that was actually the driver to come up with a bit smartly later, right? So in a nutshell, that's actually the story behind the company.
Gavin Bryant 05:50
Fantastic journey, One of the things that I wanted to dive in on explore a little bit more is those early days at Booking.com and you mentioned that early on, there was yourself and a colleague who were experimenting on the website. Now was that quite a natural, organic process that the two of you identified an opportunity to start experimenting on the website, or was that a top down initiative to begin with?
Jonas Alves 06:26
Well, it came from different ways. First, as I mentioned, I try entrepreneur and entrepreneur [phonetic 6:34], we built an internal platform, an in-house tool as well, but it was like the most expensive infrastructure that we had in the company, more expensive than the website and everything else right to aggregate all the experiments. We were not a big company, and we didn't have a lot of traffic, but because we wanted to have insights in real time, the aggregation infrastructure was heavy. And we thought, well, maybe nowadays--- This was 2018 maybe 19. I think it was still 18. Maybe nowadays, experimentation platforms are better. And let's look at a third party that maybe can do this in a cheaper way and then we don't have to develop to continue developing our own tool. So we look at Optimizely, a bit ACM, a bunch of third party tools, and none of them was close to what we had built in-house. They didn't have the same features, and the quotes were crazy expensive. So we thought, well, maybe we should actually think about coming up with a company that does this because we did it in-house, and also I had done it for a ton of times for different customers. So I thought, well, maybe that's something that we should actually do and help other companies do this in a better way because it seems that there's this gap in the market where the tools that exist are more focused on marketing, basically, so not product teams that want to run experiments holistically across organization, but more on basically by passing developers so that marketing can run experiments. So that was the focus of most tools. And what companies need is something different. If they come from a product, if they are product led so if they want to run experiments in the product itself, most of the times we were building it from scratch. So basically, those were the two angles that we had that we thought, created that gap in the market.
Gavin Bryant 09:00
Just getting back to the early days at Booking.com, I've heard you say on other podcasts before that the A/B tests that you were conducting in those early days, 2008, they were unsophisticated, they were simple, they were uncomplicated. But you had some of your biggest wins and biggest gains. And also being mindful that the booking.com website at that point was unoptimized from your experience that nothing really beats a discipline, a thoughtful, trustworthy and reliable A/B test, and we should be focusing on doing the basics first, rather than jumping ahead and trying to get too complicated. Do you think that sometimes teams and organizations they maybe get a little ahead of themselves, rather than just focus on doing the basics right, and doing the basics really, really well?
Jonas Alves 10:07
Definitely, I think that many companies over complicate stuff, and sometimes they try to start with experimentation without having the simplicity in mind first. So if you start running experiments and it's difficult to set up a test like, if you don't make it super easy for teams to get started with, then they do not like it like they'll try to avoid it at all cost. If experimentation is not easy, if it is not part of the culture, if they are not eager to learn and try out stuff, and if they just want to build what they are meant to build and put it in production as soon as possible. They are going to try to bypass it completely because first, it seems it's complicated to set up the test. It takes a lot longer to run one experiment than just shipping the code right away, which, by the way that if you do it right, it's the opposite. If you make it super simple to set up a test like it's just a feature flag, you just go to the code, you put the feature there. Actually, it's a lot faster to start one experiment to gather insights then finish the product, the feature from end to end. Test it, do quality assurance and put it in production when it's completely ready. Then it is to start it writing sooner and start learning sooner if that is helpful for you or not. You can come up with a pretty quick test from the beginning to learn if that feature will be helpful for your users, and then started interacting on that very quickly. As long as it's super easy to set up experiments that should be the basic like make it very easy to set up a test. It should be just an if-clause, if this is running, do this. So developers and designers should be able to just go there and start running experiments without any effort, and by doing that, if they started writing code to learn faster, of course that you reach the end goal and the feature in the end is much better than what it would have been if you just build it completely from end to end, right away.
Gavin Bryant 12:45
One of the things that we've talked about on the podcast previously is when the human cost of experimentation becomes too high; it starts to push people away. That if there is too much friction, if it is too abrasive, and if it is too difficult, then teams and people can really work around experimentation. One of the things that I wanted to touch on from booking.com days, Jonas, the top three learnings from your time at Booking.com like three things that you've taken with you throughout your career. You started the program from scratch, you built the platform, you established metrics, statistical principles, analysis, and also conducted extensive training and development with teams from that really broad and holistic experience of taking a program from zero to performing hundreds of experiments per day and 1000s of experiments concurrently. What are your top three things that are really important to you from that experience?
Jonas Alves 14:06
I think maybe the first is what we just talked about, that things should be simple. If you are over complicating it, making it super difficult to set up an experiment like some tools require you to add a configuration in the code for the experiment. Then you need to go to the tool and start the experiment with that idea of the configuration. People will skip it. It's too complex to get started. So they'll never go far with something that is complex. You need to make it as simple as possible so that's the first thing. Second one is that is communication across the team. The Dutch are very direct and in the beginning, that was super difficult for me. I thought, well, my boss doesn't like me, he's always telling me that I'm doing stuff wrong, the things wrong but I ended up loving it. The feedback at the moment direct, no, this is crap. Like, you should try it again. This is not good. It's awesome. You get early feedback right away. But I love that approach and that's I think it's very difficult for Latins to be like that, but I brought that with me, and I try to use it as much as I can right being like giving early feedback and right away, being direct about stuff, about your intent. I think it fixes so many communication issues, not only in organizations, but at a personal level everywhere.
So we should learn a bit with the touch on that, and for the Latins, that's actually not easy at all. Another one is like people are usually so much afraid of running many experiments of interactions and stuff. It's something that almost never happens. It's not a problem to run dozens of experiments simultaneously. Like at booking, we're running 1000 or sometimes more than that. As long as you are sure that experiments have well---- That you try to avoid it. You don't want to run experiments in the same button. You don't want to run one experiment that changes the CTA of the button and another one that changes the size of the button or the design of the button, or whatever. Those will most likely interact with each other, and it might even be a good interaction, like both experiments together, might be stronger than each individual experiment, but they might actually have the opposite effect and be a detractor so one example that we're always giving is if you change the color of the font to the same color of the background then you don't see the text. So that's a bad interaction. Usually, this doesn't happen because people try to avoid running experiments in the same element, but it's still perfectly fine to run experiments in the same page, and some of them will be running on the server. Others will be running on the UI. Others will be running on the algorithms. Like that's perfectly fine.
Gavin Bryant 17:39
So with interactions I pull two things out --- through very good and disciplined, rigorous planning processes, you will identify obvious interactions up front before execution. But then there's also monitoring to support the identification of any interactions that are occurring with in-flight experiments. Do I understand that correct?
Jonas Alves 18:05
Yes, and we built monitoring for interactions just because people are afraid of them because it almost never happens. Actually since we built it, I think that we had two alerts about it, and one of them was a false positive. So it's something so rare that I don't think that people should actually be concerned about it, because most of the times, people are conscious about what they are testing. And they know when two things should not be tested at the same time. So interactions happen when they are intentional and that's fine, and you can actually start two different experiments with the intent that they will actually interact with each other, as long as you have a way to look at them together as well. And that's why, at ABsmartly, we are planning to build a combined report. So you can run two different A/B tests and then combine them into a single MVT. So you can look at all the combinations of all the different variants of the different experiments, and look at it in a single report to see what is the best combination and the worst combination of all the variants together. So as long as you have the intention, then you know that you are actually building for an interaction. So you have two different feet. It's basically an MVT test. If you just put it together, then you can look at all the combinations and see, hey, what's better, what's worse as long as you have that ability, then it's perfectly fine to intentionally create experiments that will interact with each other, but most of the times, interactions just don't happen. Like, if you don't plan for them, they don't happen. And if they happen, it's nice to have an alert about it, just to peace of mind. But it's not super important. If you start without it, I don't think it is important. We built it only for peace of mind and because people are concerned about this all the time.
Gavin Bryant 20:05
That's a really interesting data point that you mentioned there. There was effectively one interaction identified in the many, many 1000s of experiments that were being performed concurrently, so that the likelihood is very, very small if managed correctly.
Jonas Alves 20:22
Yes, well this example that I gave about one false positive and two events and one was a false positive was now at ABsmartly. At booking, I think that we ended up not having the alerts because most of the times were false positives. So what we did was to ask people to if they thought that there was an interaction, they would look at it because with 1000 of experiments running, the majority of the alerts were false positives. Almost never was a real one. So we just didn't have the alerts going on there. But at ABsmartly, now we do it in a smarter way so we don't have that many false positives anymore.
Gavin Bryant 21:08
Our audience and listeners can breathe a big collective sigh of relief, then to just relax when it comes to interactions. A final question I wanted to ask you, and I quite enjoy asking this question of all of the guests, what's your strongest held belief about experimentation, what's the one thing from your experience that you see to be true?
Jonas Alves 21:48
My strongest belief about experimentation is that you should actually use it holistically across the organization. Most organizations use experimentation, basically in marketing and maybe in product if they're a bit more sophisticated, but most of them start just in marketing, and it's something that you should actually use across the organization. Of course, that where it helps the most is in marketing and products but you can run it in operations. You can run it in customer success. You can run experiments pretty much everywhere. At booking, we run many successful experiments in customer care, we had at the time, a bunch of people working in customer support, we had 4000 people or something. So we even would build groups of agents, we would separate them in 2000 people in one group, 2000 people in another group, and we would change the UI, like how they would pick up the tasks in the UI to see what was the one that would improve the processes the best. So they had multiple metrics at customer service, like they had the number of time spent on the calls, the number of tickets taken, so on so we would improve those metrics by changing the processes that they had internally. So you can run experiments everywhere. You can run experiments in your infrastructure. You can run experiments in your micro services, like so much that can be tested. You can test pretty much any change that, or not only on a technical level, but also on the process level, any change that you do can be tested.
Gavin Bryant 23:47
That's one of the things that I'm very bullish on, is that there will be a big shift away from, I guess maybe more of a singular focus at the moment, on experimenting on web and mobile, and that experimentation to your point and the examples that you just provided will become much more broad, and it will touch on many different value pillars and parts of the value chain in a business in the future. Let's flip the conversation now and let's have a chat about ABsmartly. One of the questions that I wanted to ask you was what inspired you to start ABsmartly, but we touched on that a little bit earlier, and you provide a really good overview of the gap in the market and the inspiration for the business and the product. So what does success look like for ABsmartly going forward? And how will you measure success?
Jonas Alves 24:59
I think it's about empowering our clients to cultivate a robust culture of experimentation within their organization. This is actually difficult to measure, but we look at the kind of questions that they ask us in the Slack channel, we offer them training to focus on improving the experimentation culture, and that hopefully is helping them get better and better. Actually, we see sometimes that after training, like questions that they ask are actually better than before. So we try to help them with that. Secondly, it's about the adoption and the impact of our platform in their business as well. So, we look at metrics such as the number of experiments that our customers run, the overall value that they can derive from the experiments that they run. We look at the positive feedback that they give us. We try to create long term partnerships, and like to see them achieve their goals. So if we see that they achieve the goals that they are meant to achieve. That's a good indicator of success for us as well.
Gavin Bryant 26:15
Thank you, and your company that has an experimentation platform and helps your clients and your customers to become more successful with experimentation. So how do you apply experimentation at ABsmartly? How do you learn?
Jonas Alves 26:34
Yes, actually recently we started running AB tests ourselves, like we always wanted to do it from the beginning, but we were a bootstrap company, we had zero customers. In the beginning, it was just two people building the product, and I was mostly trying to sell it, even without having the product ready yet. And Marcy was building the product, and actually he made an amazing job, like in six months, he built the platform from end to end but we had done it before, so we had some experience with it, but only recently we were able to actually start running experiments in the website. So we started the first experiments, but the traffic is so low and we don't have features that we are adding to the website because it's still early days for us as well. Something that we want to do, and we are planning to do that soon, is to start using feature flags in the product itself, and then later real experiments in the product. We don't have a lot of customers, so we will not have a lot of data, but basically what that will allow us to do is to go faster, ship product faster because we can mitigate risks in a much better way with feature flags and with experiments, even if the metrics are more focused on the quality of the product than on the business. Because then we can actually make positive decisions. If we look at business metrics, would not be able to make a decision in years. It's a B to B product, we have very few customers and very low traffic, that would be impossible, but I think that people can run experiments without a lot of traffic as well, as long as you focus on the right things and for us, it's more about the quality of the product, like not having errors, not impacting the load times of the platform and so on. So if you focus on quality metrics, then you have much more power to make decisions,
Gavin Bryant 28:47
And no doubt, supported by lots and lots of qualitative customer feedback and insights.
Jonas Alves 28:54
Exactly so that's something that we always try to do, is to actually work together with our customers to build the new features as well. So we ask for feedback, we send them the mock ups. We try to understand if that would fix the problem for them before we actually build it. So I think that's a very nice interaction that we have with our customers as well.
Gavin Bryant 29:16
Yes, that's really, really interesting, that customer co creation process effectively.
Jonas Alves 29:23
Exactly.
Gavin Bryant 29:25
We talked about this a little bit earlier, that your strongest held belief that your one universal truth was that experimentation is a broad based utility that should be applied holistically across business so apart from more experimentation being conducted in more verticals within a business and with more teams and departments. Is there anything else that sticks out to you around what the future of experimentation in business will look like?
Jonas Alves 30:05
What do you mean, like what is the future of experimentation in organizations?
Gavin Bryant 30:10
Yes, we touched on that we expected to see it keep shifting further and further away from a digital first basis so a lot of experimentation at the moment around web and mobile. But to your point that there's no reason that teams can't be experimenting in operations teams and in customer service teams and lots of other teams. Is there anything else, apart from the expansion of experimentation into other business teams that is really top of mind for you at the moment? Anything else that sticks out?
Jonas Alves 30:51
I think that's the most important one so basically expand it across the organization. I think that advancements in technology and AI will also facilitate many things like the real time data analysis, more sophisticated statistical methods, greater customization. So you can very easily embed it in pretty much all the tooling that you have and have everything be able to be automated with experimentation basically, because I think that the future is that all the software will be API first. Now with AI and with agency, you want to be able to have agents plug into stuff and do stuff on their own. So I think that it will be a lot easier to run experiments everywhere, because you can just plug to the API and do whatever you'd like. So it will be a lot easier than it is now to run experiments in whatever place you need. I think that it's still crucial to create a strong culture of experimentation across organization. This means that leadership needs to support those efforts from top down, and also you want people to start bottom up, coming up with ideas on how to make it better as well. So basically create continuous learning across the organization and encouraging teams to collaborate with each other in a better way and share results and share ideas across the company. Actually, this is something that I always thought, that it is crucial in experimentation, that social aspect of experimentation, of sharing ideas with even outside of your teams because something that might have worked for your team might actually be very good for other teams as well or it might create ideas so that sharing of insights, of learnings, creates a culture across the organization that helps everyone. I think, yes, that's mostly it. And who knows if humans will still be in the loop, or if at some point, AI will take it over. I think that for the next years, we are still safe, but let's see what the future brings us.
Gavin Bryant 33:27
Yes, that was one of the questions that I want to ask you. So let me jump forward to that is what role will humans play in experimentation in the future?
Jonas Alves 33:39
Yes, I think that humans will still be important for their creativity and making decisions. I think that AI will help a lot. Many things can be automated. They can probably give insights. They can do the analysis automatically, make decisions on their own. But I think that at least for the next year, maybe at some point, I don't know where things are going but in the first years that humans are still critical to--- Like, critical thinking, to create a nice hypothesis, to be creative, and maybe think about different ways to go in the organization because AI will optimize what exists now. But you need to have a strategy, and you need to think what are we going to do next and that's something that AI might be there at some point, but at least for a few years, you need humans to do that kind of stuff.
Gavin Bryant 34:49
Yes, that's interesting. You mentioned two key things there that creativity and creative problem solving, but also playing a role in the interim of the medium term around governance as well and potentially decision making, and it'll be really, really interesting to see if we get to a point with AI and businesses that are brave enough and willing enough to give AI the keys to the bank and let it operate unsupervised, or humans will always have that crucial decision and governance oversight.
Jonas Alves 34:56
Exactly.
Gavin Bryant 34:56
Now, let's talk about some opportunities for organizations. You get to work with a lot of organizations, a lot of different experimentation teams. What are some of the opportunities for organizations, and what are some of the common mistakes that you can share with our listeners and audience so they can potentially avoid them?
Jonas Alves 35:59
One of them is what we mentioned, like being afraid of interactions like that's a big mistake. They don't happen, right? What else comes to mind, the interactions is a big one. I think that what we mentioned in the beginning, like over complicating things, like, it's something that really detracts teams from experimenting correctly. You should have something that is really easy to use. It should be super simple to set up experiments, should be super simple to make decisions about experiments as well. Basically, self-service is quite important. If you need to have your data science teams aggregate the results and create a custom report every time that you run one experiment, you will not go far so teams will have to wait one or two weeks to see the results of your tests. So basically, they'll get motivated by running experiments if they have to wait two weeks to know if the experiment that they ran was good or bad. You need to give teams tools to be able to do stuff on their own and communicate with each other in a nice way as well, right? So I think that's one of the big mistakes, is that they make things too difficult to experiment, to make experimentation embedded into your development processes, basically. So I think those are the most important ones. I would say, make it super simple to experiment. If it is too complicated, people will not do it. Don't think about running too many experiments. That's not an issue at all. Yes, that's what comes to mind at the moment.
Gavin Bryant 38:09
So thinking about the future for ABsmartly, what's next for AB smartly? What are some of the exciting developments that you're exploring at the moment?
Jonas Alves 38:21
Well, first, we want to help our customers build the right culture of experimentation. So we are working a lot on building tools for that. So we are working on a dashboard for the experimentation program, where teams can see how the experimentation program is evolving over time and how it is helping the company. So you can see the impact of all the experiments on the business quarter over quarter, week over week, month over month, and see how it is improving the business metrics, but only the quality. So you can see, hey, are we increasing the number of bugs in our experiments or not? When are we restarting too many experiments because of issues with quality? So they can have visibility across the whole experimentation program. So that's something that we are very excited about. Because most tools focus on individual experiments, and the most important part is actually looking at experimentation program as a whole and I think there's usually disconnect between experimentation platforms and businesses because of that. Because they focus on individual experiments, instead of looking at it as something that is overarching about the business, the insights, the learnings, the velocity, all of that is the most important part. And yes, there's not a big focus on that, on giving visibility to make decisions globally.
Gavin Bryant 40:11
One of the things that really stuck out to me from reading Aleksander Fabijan's paper, ''The Experimentation Flywheel'', which he authored with Lukas and there was a little flywheel in the paper that the value investment flywheel, and what you were talking about there, it really reminds me of the value investment flywheel, and what you're seeking to do with clients is to surface up all of the benefits of the program, which then also feeds into cultural development and also business values, so which then feeds more investment, and the cycle just keeps going. The more value the business gets, the more they invest, and it helps that program to really flourish, grow and expand. So I think that's a really important little flywheel to be mindful of, for programs.
Jonas Alves 41:21
Exactly, yes. This is super important. At booking in the beginning, as I mentioned, we could only see the conversion rate, and we could run very simple experiments, but we saw the results, and then we could invest in building better tooling to give us more insights and run more sophisticated tests. So it just fits on itself, it's amazing.
Gavin Bryant 41:47
Let's finish up with our fast four closing questions. So these are just four quick, fun questions to finish off with. So number one, what are you learning about that we should know about and think about a personal example and something that you're learning about in the experimentation space?
Jonas Alves 42:08
Yes. Well, lately as expected, I think delving a bit into AI, learning how we can use it to become like a more efficient business. How we can use AI to make our platform better as well. I think that we can probably use it to give better insights about the experiments that were run, about learnings that people didn't notice in experiments, or even just to help you write better hypothesis so that's super easy to do, and that's something that we should explore more. On a personal level, I'm embedding myself. Well, I've been doing this for a while, learning more about energy arts and meditation and mindfulness, and overall well-being. I think this journey has helped me improve my physical health, and not only the health, but also the creativity, the focus. I think that doing this really helps, and that's a profound impact on personal and professional aspects of life.
Gavin Bryant 43:26
Keeps you grounded and keeps you present.
Jonas Alves 43:30
Yes, exactly and with the better energy as well. You can be more focused. You can work better in---
Gavin Bryant 43:39
Yes, increase productivity.
Jonas Alves 43:42
Exactly.
Gavin Bryant 43:43
Number two, what's been your most challenging, personal struggle with experimentation?
Jonas Alves 43:56
So maybe it's not really personal, it's more and more an example from the booking times was when we were growing so fast, like booking, at some point, was doubling the amount of developers pretty much every couple of years. And that was a struggle, because the culture of experimentation was disappearing, was getting diluted, because we had so many more new people than the old ones. So a big struggle for me was to keep people trained. So basically, at some point, I was training people every week so every week, I was leaving a training for new hires, because every week, we're having new developers and designers and so basically that was the biggest effort that I had. The biggest struggle with exploitation was to keep the culture intact in the organization and actually help that culture grow and flourish across the organization because if you don't have that, especially in growth period then people don't know what to do. They will do it in their own way, and they will not learn with each other.
Gavin Bryant 45:07
So that's probably a really key takeaway for our audience, that as the experimentation program grows and expands and it scales up that you need to keep pace with the growth and the experimentation skill and capability development, because if they get out of step, and the growth keeps surpassing the organizational competency and skill and capability, then, to your point, it gets diluted, which then impacts quality, right?
Jonas Alves 45:42
Exactly.
Gavin Bryant 45:45
Number three, what's the biggest misconception people have about experimentation?
Jonas Alves 45:49
I think the biggest one is that experimentation is for marketing only. Well, that's changing now. I think that we are seeing more and more organizations focusing on improving their own product with experiments. And that's strange because experimentation, it came from the product part. When, Microsoft started doing it in the early 2000s it was on the product, not on marketing. Booking and Google, all of those big companies that started running A/B tests very early, they were improving the product. But when the general rule started experimenting was mostly because Optimizely came up with a tool that was--- Even before Optimizely, I think there was Google Website Optimizer but it was mostly for improving conversions on a landing page. So it came from that marketing level, and even product is the next step, but is not where it happens. I think that people still need to look at experimentation politically, as we mentioned before. You can run experiments at a much deeper level anywhere in the organization.
Gavin Bryant 47:19
Final question, closing out our fast four, what's the one thing our audience should remember from our discussion today?
Jonas Alves 47:30
I think it is that people should look at experimentation as the default approach to improve anything in the organization. It starts with the marketing and product, and it can go more than that. Make data driven decisions to deliver better results across the board, and it allows you to focus on growth and improvement. So you can basically do faster decisions by trying out ideas and see which ideas work. And you can do that at many levels not only with A/B tests, but with many different things like you can start with the testing. Let's see if we have some traction with this idea, and if not try different one, because if people don't experiment, then they don't learn as fast. If you just ship stuff and you go with the first idea that you have, you just don't learn as quickly. You go much slower so basically, be data driven, be willing to test stuff, even it doesn't have to be an A/B test. You can run one experiment just by trying different ideas and see which one works best. I think that's something that people usually don't give a lot of importance on, and it's crucial, like, it's the mindset, the mentality of just trying to learn more.
Gavin Bryant 49:02
Yes, I agree, and that's a fantastic way to finish. I completely support and advocate that point that I've been a big believer in that the experimentation is a broad based utility that should be applied right across a business. And it's not completely limited to just digital domains so great way to finish Jonas. Thank you so much for your time today. Really appreciate it. And again, thank you so much for your support for the 2024 Asia Pacific Experimentation Summit. We're really looking forward to the event in four weeks’ time. Thank you.
Jonas Alves 49:42
It was a pleasure to be here speaking with you. Talk soon.
“ When you start experimenting, if it’s difficult to setup a test people will avoid it at all cost. You need to make it super easy for teams to get started. If experimentation is not easy, not part of the culture, and people are not eager to learn and try new opportunities, they will just launch their change into production. If experimentation is too complicated teams will bypass it. You need to make experimentation easier than shipping code ”
Highlights
Jonas joined Booking.com in 2008. The organisation had been performing experiments since 2005. The Booking.com experimentation program started small, growing organically. Initially, it was Jonas and a Designer performing all of the early experiments. They began by performing simple A/B tests. These early tests produced some of the biggest successes for experimentation at Booking.com
Jonas transitioned into the Head of Experimentation role at Booking.com. He was responsible for building and developing the experimentation platform, training and capability uplift, statistics, metrics etc. By 2015, Booking.com had become an experimentation factory, performing 100-200 experiments in production every day, with more than 1,000 experiments running concurrently across the website
Start with simplicity in mind - many companies overcomplicate experimentation, making it too difficult. Experimentation needs to be as simple as possible. If experimentation is too difficult teams will bypass it, shipping their change into production. You need to make experimentation easier than shipping code
Relaxing about experiment interactions - the ABsmartly experimentation platform has inbuilt monitoring and detection for interaction effects. Teams are afraid of experiment interactions, however, they are very rare. Across all customer experiments performed on the ABsmartly platform there have only been two alerts triggered - one a false positive and the other a legitimate interaction. Interactions are so rare that people shouldn’t be concerned about it.
Strongest belief about experimentation - experimentation needs to be used holistically across an organisation. Experimentation should not be limited to Product and Marketing functions. Experimentation can be conducted in Operations and Customer Support. Experimentation can be applied everywhere. At Booking.com experiments were conducted in Customer Support, splitting agents into Control vs Treatment groups to test UI to improve process efficiencies. Service orientated metrics (Time on Call, # of Tickets Completed) were measured
What does success look like for ABsmartly? - empowering clients to develop a robust culture of experimentation in their organisations
How does the ABsmartly team experiment on their product? Testing on the website is challenging being a B2B product - traffic is too low. In the ABsmartly platform, the team will start using feature flags to experiment and mitigate risk, enabling the team to move faster and ship product faster. ABsmartly work in very close partnership with customers, using qualitative feedback and co-creation to build new features
Advancement in Technology & AI - will facilitate real-time data analysis, more sophisticated statistical methods and greater customisation. AI can potentially be embedded in all tooling. The future of software will be API first. Experimentation will become easier and more accessible plugging in to an API’s
Creating a strong culture of experimentation is crucial - Top-Down leadership is required to support strategic experimentation efforts, while Bottom-Up support is required to make experimentation better. We cannot underestimate the role that social hierarchies play in an organisation to collaborate, share insights and learnings and create a culture of experimentation across the organisation
What role will humans play in experimentation in the future? In the short to medium term humans will still have an important role to play in experimentation - generating creative solutions, critical thinking, hypothesis development, decision-making etc. Humans will have a key role to play in strategy development and prioritisation - to ensure that experimentation efforts are focussed on the right things to deliver maximum impact for business and customer
Top 3 experimentation mistakes 1). Worrying too much about experiment interaction effects 2). Making experimentation too complex and time consuming 3). Experimentation is not embedded into development / release processes
What’s in the pipeline for ABsmartly? To develop a fully-integrated experimentation dashboard that will provide customers with a holistic overview of the performance of the end-to-end experimentation program
Scaling experimentation training and capability uplift - when experimentation is growing and scaling out at a rapid rate it can be very difficult for the Centre of Excellence team to keep pace with experimentation training requirements. If experimentation organisational capability is not in lock step with experimentation scaling the quality of experiments can decline
Establish Communities of Practice to scale experimentation faster - If you can develop peer-to-peer communities, and teach one another, it’s a more scalable approach to improving overall decision quality than having a centralised experimentation authority. Developing communities of practice can increase and accelerate your organisational performance with experimentation
In this episode we discuss:
Early days of experimentation at Booking.com
How Booking.com became an experimentation factory
Why experimentation needs to be as simple as possible
Why you need to relax about experiment interactions
Applying experimentation across an entire organisation
How ABsmartly experiment on their own product
Advancements in Technology and AI
The future role of humans in experimentation
Avoid these Top 3 experimentation mistakes
What’s next for ABsmartly
Scaling experimentation training and capability uplift
Success starts now.
Beat The Odds newsletter is jam packed with 100% practical lessons, strategies, and tips from world-leading experts in Experimentation, Innovation and Product Design.
So, join the hundreds of people who Beat The Odds every month with our help.