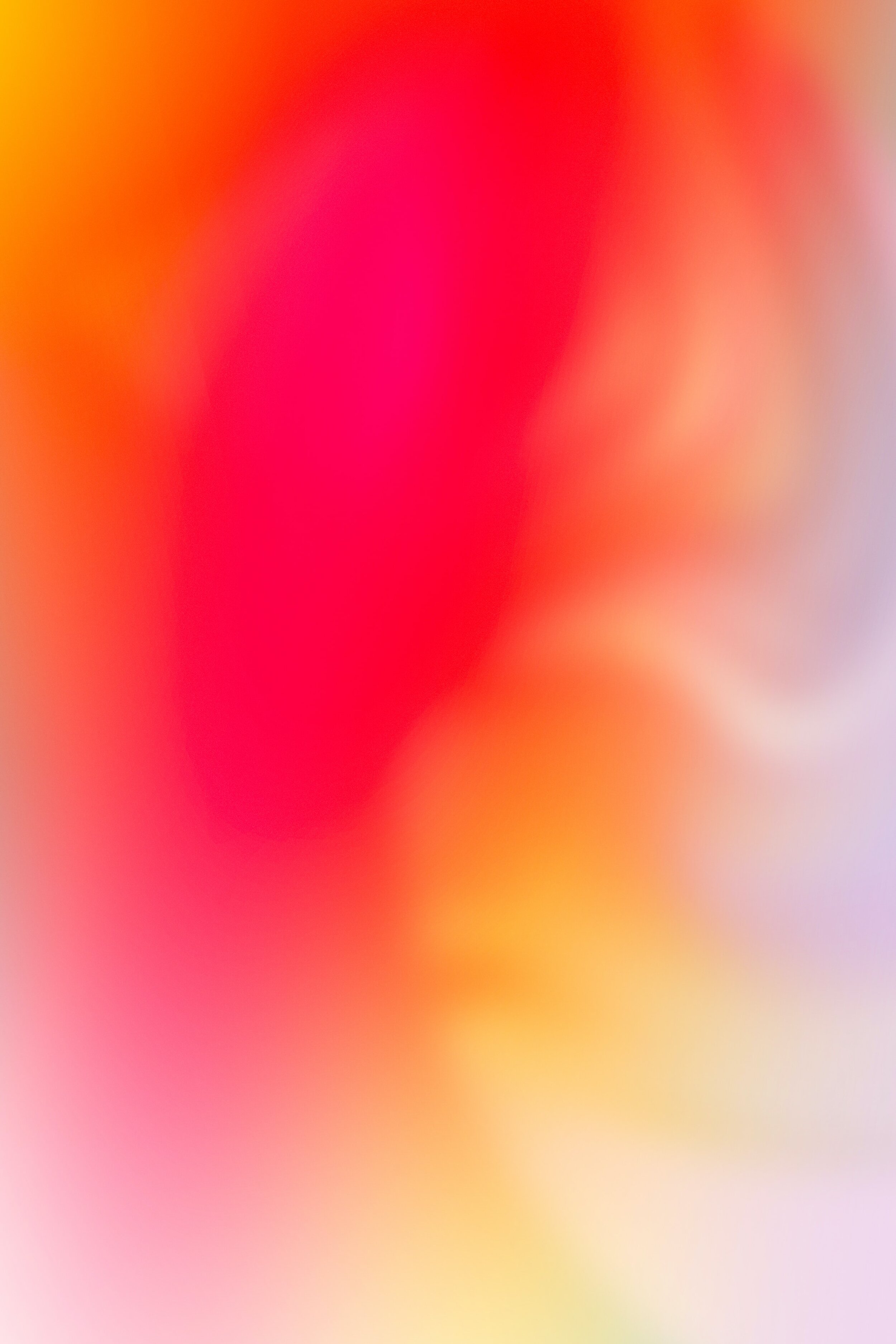
November 5 2024 • Episode 020
Ken Kutyn - Amplitude - Developing High-Velocity Experimentation
“ A/B testing is like a game of chess. It's competitive. You're up against other teams trying to take your market share who are A/B testing too. We need to throw all our resources behind experimentation. To make sure that we're keeping pace, getting ahead of more established players, and startups, who are moving quickly and experimenting, invading a lot. If we don't treat experimentation like a game of chess, where we're constantly trying new moves and new strategies, and trying to stay ahead of competition, you’re going to get left behind. ”
Ken Kutyn is a Principal Solutions Engineer at Amplitude Analytics, with over 8 years of experience at the forefront of Experimentation platforms, including Optimizely and Amplitude. Throughout his career, Ken has partnered with some of the world’s largest brands, guiding them in evaluating, implementing, and scaling their experimentation programs. His journey has taken him across major global tech hubs, including Vancouver, San Francisco, Amsterdam, London, and now Singapore.
Ken brings a deep technical expertise and passion for experimentation, focusing on enabling high-velocity testing without compromising user experience. His work helps teams eliminate performance bottlenecks, prevent bugs, and ensure clean data integrity—critical factors for successful, scalable experimentation.
After publishing his MBA thesis on the role of experimentation in software development, he has become a sought-after speaker, sharing best practices at conferences, webinars, and podcasts worldwide. His mission is to empower teams with the knowledge and tools to drive impactful experimentation and continuous improvement.
Get the transcript
Episode 020 - Ken Kutyn - Amplitude - Developing High-Velocity Experimentation
Gavin Bryant 00:03
Hello and welcome to the Experimentation Masters Podcast. Today, I'd like to welcome Ken Kutyn to the show. Ken is a Principal Solutions Engineer at Amplitude Analytics with more than eight years of experience at the forefront of experimentation platforms, including Optimizely and Amplitude. He has worked with some of the world's leading brands to help them implement and scale experimentation programs, bringing a deep technical expertise for experimentation. Ken is focused on enabling high velocity testing without compromising user experience. Welcome to the show, Ken.
Ken Kutyn 00:42
Thanks, Gavin, great to be here.
Gavin Bryant 00:46
I'd like to extend a quick thanks to Ken. Ken is one of our speakers for the 2024 Asia Pacific Experimentation Summit. So Ken, thank you for being so generous with your time and for supporting the event in its first year so really appreciative of that, and really looking forward to our discussion today. You've got really deep experience. It extends right from experimentation program strategy right through to design execution. So we'll cover a broad cross section of territory today so yes, looking forward to our discussion today.
Ken Kutyn 01:26
Yes, me too. I think it's a great initiative. The conference you mentioned, I moved over from Europe to Singapore about two years ago, and it's so diverse here in terms of different countries and where they are in their maturity around analytics and experimentation. So I'm excited to be a part of this initiative too, yes, hopefully help more people up level and progress on their journey towards being more data driven and experiment driven at their teams and companies.
Gavin Bryant 01:52
Yes, now that's a really good point that our friends and colleagues in the northern hemisphere have a really tight-knit community and great professional and personal relationships that they've developed over many years of an increasing level of maturity in the industry and through lots of supporting networking events and conferences that we don't have here in the Asia Pacific. So yes, this is the first step on that journey to creating a great community network of professionals that can learn, share and connect together.
Okay, let's get started with you. Ken, if you could please provide an overview of your personal journey and your background today. Please.
Ken Kutyn 02:40
Yes, absolutely. I was living in San Francisco in the barrier, working for Oracle in analytics and their BI products, and I didn't know much about AB testing or experimentation until I saw a job opportunity at Optimizely. And like most people, I thought, I've heard this mentioned. I know Google does a bit of this, but it's relatively new field, and the more I kind of dive into it and realize there's the tech side to it, there's worrying about principles of UX and conversion rate optimization, there's the math and statistics side. I thought this sounded like a really cool product and really cool kind of solution to what many teams were struggling with in building sites and apps at that time. And so, yes, I took a jump into the kind of this new area and learned a lot working for Optimizely moving back to Europe with them, and living in Amsterdam and helping companies across Europe, yes, ultimately build and scale their experimentation programs. And obviously the time when someone is picking a new tool or new solution is often kind of a major step change in their organization, when there are some other changes going on and they're deciding to really double down on how they experiment. So that's an exciting time to be involved as someone's going from either zero to one, from nothing to their first experimentation solution and really trying to launch a program. Or they're moving from something, maybe in house that's working, but not giving them quite the scale and velocity and uplift they expected to trying to get a bit more professional and ambitious with what they're doing. Or maybe it's a new team structure or a new executive that has kind of prompted to change but regardless, it's an exciting time to be involved in a company's journey when there's a chance for them to improve their processes and strategy and their tooling and kind of kick off a new velocity of testing as well. So I work for Optimizely in a solutions engineering role, so helping people through that journey for about four years, and then almost four years ago, I joined Amplitude, and I didn't actually know at the time, they're about to launch an experiment product. My very first day of the company, and my manager said, By the way, this might be interesting to you. Here's what's coming out in a couple months’ time. And. So yes, I was able to see that journey for a SAS vendor as well, to launch an experiment product and to help brands and actually do a lot of help internally as well, with up leveling our go to market teams, our strategy teams, our sales teams, on how to talk to customers about experimentation. And it's different. It's different from an analytics product, and you have different stakeholders. You've got different factors they care about in their decision process. So yes, I've been in aptitude for about four years. Moved to Singapore about two years ago, and really enjoying it, really enjoying here, really enjoying the company and the role, and, yes, I think it's still, there's still so much good work to be done in terms of helping companies build their programs and get them off the ground. Everyone's a different maturity, like I said. And there's always progress to be made.
Gavin Bryant 05:58
Yes, awesome summary, I'm with you on that, that the industry is still young and still evolving, and the next 10 years are super exciting.
Ken Kutyn 06:11
Yes, for sure. And with everything that's going on with every tech trend and SaaS trend and CRO trend, there impacts experimentation whether it's things like data warehousing and AI and yes different movements in the cloud world like everything changes how we run tests and what types of tests we run.
Gavin Bryant 06:35
One of the comments that I'd read in doing a little bit of research for this chat today was something that you alluded to a little while ago. AB testing is like a game of chess. Could you expand on that a little bit for our audience?
Ken Kutyn 06:52
Yes, I guess something I've said in the past one conference I spoke at was like we don't know. I guess our situation as companies, whether you're running an ECOM shop or a travel website or a stock trading app, your opportunity at this point in time is unique in the sense that your app is not the exact same as your competitors. The people you sell or market to is not the exact same. No one's done exactly what you're trying to do before. And so yes, you can, you can try and copy some industry leaders, but ultimately, you need to figure out for yourself and test all of your assumptions about, how should we onboard people, what price should we sell to? How do we package our product? What do we call this feature? All of those things we need to determine for ourselves, because there's no manual that gives us the hard, fast rule. And so I say AB testing is like a game of chess. It's competitive. You're up against other teams trying to take your market share who are probably AB testing. And we need to throw all our resources behind this to really make sure that we're keeping pace, getting ahead of more established players who might have more resources to throw this at other startups, who are moving quickly and experimenting, invading a lot. And if we just--- If we don't be like a game of chess where we're constantly trying new moves and trying new strategy and trying to stay ahead of competition, we're going to get left behind.
Gavin Bryant 08:20
Yes, I think that's a couple of really good points you make there. One of the things that I liked was imitation, potentially being dressed up as innovation, that my customers are not your customers, and vice versa. So while it's good to learn from our competitors and to look at practices that have potentially worked for other organizations. We ultimately need to test those for our organization, our product, and our customers. So I thought that was awesome. And also one of the other things I've been thinking about, yes, the metaphor of chess, and also I like poker for experimentation as well, because we're taking bets, there's a lot of strategy and a lot of decision making as well. So I like those three elements as well, and also competition, as you alluded to.
Ken Kutyn 09:17
Yes, I hadn't thought about the poker angle, but that's pretty good as well, in the sense that there's uncertainty in terms of--- The cards are coming up next, our competitors hands and experimentation is it's, do we want to spend dev cycles and visitors on our website to fill in those uncertainties? And how long are we willing to wait to. We can wait longer to learn more, but it might be too late. The opportunity might have passed if we wait too long. So I think that's a good parallel as well.
Gavin Bryant 09:51
Okay, next question I'd like to ask you, and one that I'm always intrigued to ask guests on the podcast, what's your strongest held belief about experiment and why?
Ken Kutyn 10:02
Yes, that's great question. I think what I come back to is--- I often encourage teams like some experimentation is better than none, and I know this field can get sophisticated quickly when we talk about the technical concerns of testing, when we talk about the statistical concerns, and I'm the first one to admit even that gets over my head, depending on how deep we get into it. But ultimately, I think that we don't want to be scared off, and as an industry, we should be yes, pushing the envelope forward and what we can do and complex statistical techniques that give us more robust and certain answers. That's great, but let's make sure we're not discouraging people from running their first AB test, because it sounds crazy, it sounds difficult, because yes I still believe that if you in good faith, set up a test, pick a metric, read a couple blogs about experiment design, and run your first test, you're still better off than if you just guessed which version of your pricing page would outperform. And maybe you'll make some mistakes along the way. And I guarantee you'll learn throughout the process. You might not find a statsig uplift, but, but doing it is learning, and I think that still outweighs other means we have of making decisions.
Gavin Bryant 11:23
And through your experience, often, there's a position within the industry that if companies don't have sufficient traffic size, that AB testing is just not for them. What's your experience with that? And do you have a position on that?
Ken Kutyn 11:42
Yes, definitely, there are issues with low traffic, and you have to be careful. Obviously, I've worked at B2B companies for the past 10 years, so I've seen firsthand some of those struggles. Some of the things are our product growth teams would love to experiment on but are just a little bit limited. And I think that---Going back to my last question, where I said, don't worry too much about the in depth stats, if you understand the basics of the stats, you can start to pull the right levers in order to run tests on lower traffic sites. So a simple example is that most people overlook is the baseline conversion rate. So the conversion rate of your control of your unmodified site has a massive impact on how many visitors you need to reach statistical significance. So if your baseline conversion rate is 2%, 2% of people make it from a landing page to a purchase, really hard to measure an increase to 2.1% but if your baseline conversion rate is 30% because 30% of people make it from add to cart to checkout page. That's much easier to measure the increase from 30% to whatever the equivalent is. I guess it's a 5% uplift. So by picking the right pages, the right use cases, you gotta make more impactful changes right by choosing the right metrics where you've got a higher baseline conversion rate. These things are doable, and you can sit with any online A/B test sample size calculator, and try pulling those different levers. And hopefully, you'll find some use cases that do work for you and your brand and your site.
Gavin Bryant 13:20
Yes, that's a really good, pragmatic approach. Let’s shift the gear of our discussion now and work into a more practically focused discussion. One of the things that I wanted to investigate with you is more from a strategic perspective and from your experience looking behind the curtains on a lot of different experimentation programs across the world with a lot of leading brands. What does a good experimentation program strategy look like?
Ken Kutyn 13:46
Yes, I think there's a quote I keep coming back to from Lukas Vermeer, who I think is actually also speaking at the conference. Right? This one's been rattling around in my brain for a few years. I don't remember when he first said it, but he said, to decentralize decision making, you have to centralize the process and the technology. And I love this one because, yes, companies get stuck where they're like, we let all the teams do whatever they want, and now the results all over the place, and nobody trusts them, and we're all talking about different version of the numbers and the metrics, but I thought that's what we were supposed to do with AB testing, was let all the product teams be self-sufficient and enabled and empowered to make their own decisions like Marty Kagan would write about, right? So I think where Lukas gives great guidance, you got to standardize the process. You've got to agree what's the best in class approach to this that we're all going to try and follow. Let's give all the teams the right analytics experimentation tooling in order to follow those. Maybe initially we have to give every empowered product team some data science resources or analyst resources to guide them along that path until they're up and running by themselves, and then, yes, eventually we will have agreed upon these metrics. We'll have shared definitions of how to capture and calculate that data, and now we can trust the teams to go and do their own thing, make their own empowered decisions, understanding that we're all working on the same data. We're all working the same process, and if a different team reran this experiment a week later, got a reasonable degree of faith that they would, they would reach the same conclusion. I think that's something Booking.com actually encouraged teams to do, is rerun test quite often. So I think that's a good one if it's going to kind of sum it up in one cornerstone for experimentation strategy just trying to centralize the process and tooling, decentralized the decision making.
Gavin Bryant 15:44
Yes, great quote from Lukas. One of the areas that I've seen you write about previously was around high velocity experimentation and the critical success factors. So we just touched on some of those elements just then for more broadly strategic access of a program. What are some of those critical factors you see in your work for high Velocity experimentation?
Ken Kutyn 16:12
Yes, I think first of all, the importance of high velocity experimentation. If you do this right, you need to throw some resources behind it right and if you're running a test here and there, because your team takes a while to get an approval and for design to throw it together, and engineering resources are already scheduled for the sprint, it can be pretty tough to get ROI and then the program stalls, because you need a winner every test in order to justify the time and effort you're spending on this. And that's not a healthy approach. And all of a sudden, senior leadership starts looking at this like, hey, well, you're not delivering more than a winner every six months. How are we possibly getting value back from this? So, yes definitely, we need to up the velocity, something that the Jeff Bezos of the world speak a lot about, how do we run so many experiments? And I think that, like you said, it ties in a bit to the last question, centralizing the process, decentralizing the decision making. And I think that teams who are really data driven do this the best, because they've got the solid foundation. And in my job, I have people coming to me all the time about like, oh, which experimentation product is best? And I would love to sell them whatever I'm working on. But I always say to people like, you got to figure out your analytics foundation before you can worry about experimentation if you don't have trust in the data, given eliminated discrepancies between the product team and the marketing team are talking about, if you can't go up to the C level with the right numbers, the metrics that everyone else in the org has agreed upon, then you're going to struggle getting experimentation off the ground, because every time there's a test, there's going to be four people who stick up their hands. Stick up their hand and say, I don't trust this result. Where is that coming from? Where is this coming from? That really stalls things and coming out of that when that foundation is in place. Now you can do things like make analysis of experiments self-service. I have a product team do that instead of open a ticket with the data science team and wait three weeks for an answer that stalls your experiment program dead in its tracks, and now you're not benefiting from the uplift you just measured for at least three weeks. It might not even be uplift anymore. Behavior changes so I think get that foundation in place of data driven culture data informed a place where you're allowed to make mistakes, where you share learnings, not share wins, and get the teams whatever they need to make their own decisions in order to have access to reliable data, be able to interrogate results themselves and launch winners themselves. And those would be kind of the key first steps for scaling up the velocity from one a month to five month to 10 a month and beyond.
Gavin Bryant 19:11
Interesting that you mentioned resourcing, whether it's dollars or it's people, I see that as being one of the biggest constraints and hand breaks. And from a lot of the programs that I see that people are trying to as a centralized program structure, for example, to operate experimentation within the context of their BAU roles as well. And I think at the end of the day, BAU will also always win out, and experimentation can be easily deprioritized within that context. But also another resourcing constraint that I see is development resources, and in a lot of organizations, they really struggle to get dedicated developer resources to support experimentation.
Ken Kutyn 20:06
Yes, that's a big one. And we come from 2015, kind of timing, when everyone was testing in the web browser with visual editors, and now we're much more likely to see teams opting for in code in product first approach, which needs DEV to have an equal seat at the table in terms of decision making and prioritization and how we're going to test and what we're going to test and with experimentation, I mean, teams struggle, because, like you said, if something is deprioritized, if the wrong person leaves the company, if there's a scramble for a Q4 launch, and you stop testing, you can't start testing tomorrow and immediately start getting the value back. You're always two, three weeks away from actually getting a test live, running it, analyzing it, and getting some results out of there, where now the program is delivering ROI again. So I think that lag is tough where it's never something that is going to benefit me tomorrow, unless I started two, three weeks ago or longer, probably in many cases, and that's definitely a struggle for many orgs, getting DEV buy in and of course, leadership at the exact level that kind of buy in is a big determinant of whether experimentation sticks around and truly gets lift. And I've worked with, of course more digital, native companies are a little more likely to adopt this, but I've worked with, like some more traditional work that I worked with an auto manufacturer based in Europe, and we had a product team who was dying to do more feature flagging experimentation, but kept getting blocked because every code change needed six physical signatures before it went live. And experimentation sounds like risk, especially in places like Germany, where they're used to solving problems with engineering and math. And here we're coming up saying, Oh, just test it. But what we did is we kind of tried to reposition it a bit saying, and we know we need leadership and exec buy, and we're not going to fight that, but we need to show that the leadership team that actually experimentation feature flagging is our lower risk way to launch. And the focus shifts from let's help your product team optimize such and such metric to let's give you a kill switch and a way to remove code from production instantly when you need it, and we can detect earlier when you need that. And that kind of conversation shift lets us convince a traditional conservative leadership team that this is actually better for everyone and it does meet their needs as well.
Gavin Bryant 22:54
Yes, I like the notion of the kill switch. I'm really interested to see going forward. You touched on there that digital first, and technology companies, experimentation is the operating model, and it’s just a habitual way of working. And I'm really interested to see over coming years, so large incumbent operators, where they're not digital first in their core, in their essence, to see how they adopt experimentation. More examples of the automotive company that you touched on.
Ken Kutyn 23:31
Yes and these companies are hiring Chief Digital Officers, Chief Technology Officers from smaller players to try and bring some of that knowledge over. And in the past few years, I've worked with some media companies, a couple newspapers in the UK that have been around for 100 years but they're on this journey. And like we said earlier the important thing is, they're on the journey. They're taking the steps they're learning along the way. So it's exciting to watch.
Gavin Bryant 24:01
So we've talked about a couple of things so far around getting the foundational platform correct, particularly in relation to analytics, reliable, trustworthy data. We've touched on resourcing potentially being a key constraint. What are some of the other things that you see as being the largest struggles or blockers in organizations you work with?
Ken Kutyn 24:28
Yes. Good question. I think people do struggle with the analysis of experiments right? In my role, I see this a lot, where people are running their first test. It's a new team at a company trying to experiment for the first time, and it's immediately like, oh, everyone's heard of statsig. Everyone gets that much, but it's reached statsig. Does that mean we should stop it? Or it hasn't reached statsig. What does that mean? Do I keep going? I've been waiting for a month. I've been waiting for two months, and you have to come in like, no, no, don't, don't wait for two months. So, so yes people really struggle with the basics here. And I think we as an industry can do more to kind of distill down what are the important takeaways. And so one thing I wrote a bit about was the use of confidence intervals. Not a novel idea. Obviously, I'm not taking credit for coming up with that, but it's amazing how often it's overlooked. In the sense of, when you've got a test that's been live for two weeks, for a month, and it's a big change, and you would expect to see some results sooner you've got to go to the confidence intervals to see, oh, well, maybe the difference is just not that big between the control and the variant. Or maybe it's really likely to be positive, and we're a couple days away from probably reaching statsig. So that's one that people make a mistake with, or people struggle with analysis, especially of inconclusive results, and they just completely get stalled and don't know what to do with next.
Gavin Bryant 26:07
Let's think about experimentation design more specifically. Some of the elements of experimentation design that I see teams challenged with is around customer problem identification and prioritization, and then linking that through strategically into hypothesis design, and then to be able to design and execute, to be able to test that hypothesis. What are some of the foundational guiding principles for organizations that may be a little more immature in their Journey?
Ken Kutyn 26:47
Yes, you're right. And I think people too often skip the first few stages of building a hypothesis, right? It's very easy to start with a design for a checkout page and say, Oh, well maybe this step should go first, or maybe this should be a four on two pages versus one, because you're looking at the design and you're not sure which layout is better. But I mean, like you just said, we wanted to go back, like six steps to understanding our customers, what are they trying to achieve? What are their problems? Not what's the problem I as a PM have with the checkout page? What is the customer problem on this page? What is the customer's jobs to be done and their goals? And if we can understand that through the help of things like session replay and analytics and so on and interviews, then we can come up with maybe eight or 10 solutions to that problem, go back to something like design thinking, right? Trying to think outside the box, and just put all the ideas down on paper and once we've got that, now we know we're trying to solve a real customer problem. Now we can narrow down our 10 ideas, maybe with some input from UX and from engineering to the 3,4 or 5 that we actually want to experiment on. And this is an interesting one as well, because I think too many experiments are AB tests only, a there's just a variant and a control. And I think I've seen some research on this, to say that your chance of finding statsig winners increases as you try a few more ideas, because teams--- You pick one idea and test it. They're testing the idea they maybe should have launched six months ago. They're testing the idea that CEO's idea. They're testing the most obvious solution, or the group think solution, whereas, when we've got four or five variants. Now we're testing a couple more things out of left field that maybe someone on the team suggested, who had some great insight, because they were on the support team and they were talking to an upset customer the day before, or testing an idea that someone came up with because they're using a different app from a different industry altogether that had a novel solution to some kind of UX problem. And so, yes when you have 3,4 or 5 variants, your win rates go up. And people always ask, like, oh, again, I've got low traffic. What am I going to do with this? And we can get into statistics another time. But if you're using an approach like sequential testing or Bayesian inference, where you allow for a flexible sample size depending on the observed effect, then you can actually stop the test sooner. If one of your five variants has a bigger uplift, you don't have to run the test until all five reach that SIG. So I know there's some debates on this topic, but it can be beneficial, even from an efficiency and number of tests you can run in velocity perspective, to run four variants at once, then a series of AB tests, one after the other, you learn more. You learn faster. And yeah, of course, there are resourcing considerations there as well. So to go back to your question. I think that's a good one. Go back to the customer problem. Not your businesses problem, the customer problem. Brainstorm a lot of ideas to it. Try and get as diverse perspective as possible. Narrow them down using a prioritization framework. There are a lot of great ones out there. I don't have a favorite, per se. And then try and implement 3,4 or 5 variants, if time and resources allow, and I think you'll notice an effect right away.
Gavin Bryant 30:24
I think that's a really important one, that you highlight there customer problems versus business problems that I think a lot of teams, and I observe this quite frequently, that they will observe an impact or key metric, or a decline and a fall in a key metric, and then really double down in trying to solve the movement to that metric, rather than pulling the thread and then going back further to understand what's the custom behavior that's driving that decline in metric and why did that occur, and addressing the root cause. So I think it can be really easy to get fixated and caught up in solving the symptom rather than addressing the root cause, which is always a behavior.
Ken Kutyn 31:14
Yes and I mean if you go back to what we're all trying to do, or claim to do, is be agile. The goal of agile was to just be that much closer to our customers and hearing from them as often as possible, and taking that feedback and build it into development and experimentation. It’s such an incredible way to do that at scale with statistical rigor. But if we realize we're doing things that we're trying to experiment in ways that are not connected to what the customer needs. Then the process is broken. We're doing something wrong.
Gavin Bryant 31:49
Yes, it's interesting, I guess at the end of the day, it all harks back to knowing and understanding your customer as best as you can.
Ken Kutyn 31:57
Yes, that's what it's all about. I agree.
Gavin Bryant 32:02
Hey Ken, you wrote a really good article a little while ago on five common overlook steps in AB testing, and I just wanted to pull out a couple of elements to that. And one of the things that I wanted to discuss with you, and you touched on this a little earlier, when you were suggesting that teams can sometimes be a little challenged with analysis, and one of the things that I loved about performing experiments the most is always the segmentation and diving into the segments to understand what's happening, two or three layers down, and that was driving those potential top line impacts and results. Could you talk a little bit about that?
Ken Kutyn 32:46
Yes, I think this is critical nowadays. And product teams should hopefully have done some kind of exercises where they talk about their ideal customer profile and who are we building for? But probably you don't have a single profile, right? You don't have a single type of user. And even if you do the different stages in that customer's journey, they have different needs. And so yes, ideally, after we run an AB test, we want to check that this holds true for our key subpopulations. Otherwise, we risk optimizing the app for a general user that doesn't actually exist, or one of our populations at the expense of others. And yes, you can imagine that when people are onboarding for the first time and they have different needs and expectations and goals for what they encounter in your UI versus that power user who's been around for five years. And so yes, it's great to segment for things like iOS versus Android, make sure we don't have some kind of bugs or some weird behavior, but ideally, we're getting more into the behavioral level of segmentation as well. Does this flow work for someone's first purchase as well as it works for their 10th purchase? Does it work for our power users versus our users, who we believe are actually at risk of churning because they've contacted support 10 times this month? So any kind of segmentation we can do there will just make our results that much more rigorous, make sure we're not implementing things that appear to be winners, and then we find out three months, six months later, wow, that really hurt revenue. I mean, probably we won't find out, but we'll just see revenue dropping. We won't know why. So always great practice. And the main caution there is just to make sure you're---- If you find something interesting, well, if you check too many segments that can be a false positive. So if there's something interesting you're going to act on, you might want to go back and rerun the test just at that sub segment level or do some further analysis, but it's definitely good practice to kind of make sure you're seeing the full picture of your results.
Gavin Bryant 34:49
Yes, definitely, segment analysis is the most fun. I love it. One thing that I also wanted to pull out of that article was about knowledge management and documentation that sometimes a lot of experimentation results, they can sit in people's email inbox or in multiple disparate systems across the business. From your experience, what does good experimentation, knowledge management look like?
Ken Kutyn 35:17
Yes, I wish there was a silver bullet here. And this is something I think software companies have to admit that we can help, but we can never solve, right? You look at many experimentation products have something built in to help automate a bit. You've got the like JIRA, Confluence and similar, where people document this, you get Google Docs and Spreadsheets. You get Notion and then Miro, so yes at the end of the day this is often needs a lot of ownership from a company where someone's just going to make that their passion and make that their wheelhouse, to just be a little bit annoying, maybe sometimes be a little bit unpopular, sometimes. But say this is something we do as a company. We document our AB tests, and we do it in this format, and we make sure we share them with the company in this way. And, yes, it'll pay dividends, and you'll want something that is easy to search and look back on and see the context of AB tests that have been run in the past, and that can all those things---- Just make your testing program and your future experiments that much stronger. I wish there was an easy answer.
Gavin Bryant 36:28
Yes, really good point you make the ownership and yeah it needs to be a really strong discipline of the team in the organization.
Ken Kutyn 36:37
Yes. Those are the only times I've seen it succeed, no matter how fancy their tech and their software gets, it only works when there's someone who cares about it and takes ownership.
Gavin Bryant 36:48
To the audience, I'll post a link to Ken's article about that. It's a good overview, and another one I want to discuss with you Ken, was to multi arm bandit or Not?
Ken Kutyn 37:01
Yes, this is a funny one because it's something that everyone who is a little less sophisticated with AB testing thinks they need. It sounds great, right? And we can all be forgiven for reading a summary of what multi armed bandits are and doing well. Why would you not run this every time? And for anyone who's new to the idea, I'm sure your listener base is probably quite well informed. But the basic idea is that we would change the traffic between A versus B versus C versus D while the test is running, as we see which variant is giving the most returns, the most uplift, or conversions. The idea being that, instead of running an AB test, analyzing it, learning from it, implementing the winner, we can just start to benefit from the winner immediately. And I think that's great, but I don't think this is a really a great substitute for statistically rigorous AB test. This is more suitable for a short term optimization. So we'll give an example here. You've got, depending what country you're in, maybe you've got Christmas upcoming, or Halloween in Singapore, the next is in Bali. And maybe you're running a promotion for the upcoming holiday, and you want to sell whatever item on your site, and you've got a banner, well, if the holiday is only a week away, you don't have time to run an AB test Monday till Thursday and then publish it on Friday and expect to get much impact there for a couple of reasons. One, there's only so much time left after you launch your AB test, where you can actually benefit from it. And two, maybe the last minute shopper behavior is different from the current behavior in the week or the days leading up to that. So it's not a great candidate for an AB test. So this is where Multi Armed Bandit comes in, the idea that we could have, say, three banners with different discount codes or call to action on the top of our landing page, and we can let the software, once an hour, check the conversion rates, see which is working better, and give a bit more traffic in that direction. And this is great, because it can pivot from Monday to Tuesday to Wednesday, if one of them is clearly the best by Tuesday or Wednesday or Thursday, we expect that one's getting almost all the traffic, and we're immediately benefiting. We're getting increased returns while the optimization is running. Now, where this is not a great fit is we haven't necessarily learned anything statistical. We can still apply some statistical tests to this outcome, but generally speaking, many of those have assumptions about consistent traffic allocation, and we want it to be fair in terms of them getting equal exposure at different time periods throughout the experiment, so it's not the most rigorous way to learn. For example, if we're running a change and we've got some kind of a productivity app we're making changes to the main Nav bar. This isn't something we need to optimize different from Monday to Tuesday. This is a major strategic decision that we're probably going to live with for a year. And so, yes, I'd want to run a true AB, ABCD test on it for two weeks or a month, whatever your statistics require, do a thorough analysis of it, and then launch the winner for everyone, and maybe we revisit that in one year's time. And so when you're trying to decide multi arm banded versus AB test, that's the kind of thought process I would have. Is this a short term optimization where we just need to maximize conversions? Or is the emphasis here on learning and making a decision and benefiting from that decision for some time. So I think multi embedded have some potential, some perfect applications, but I do, unfortunately see them a Little bit overhyped for being completely honest.
Gavin Bryant 40:55
Yes, I think a good, good point there to really think carefully about the use cases and the application of it. And one of the other things you touched on there was teams think they should be performing bandits. So just to reverse up on that a little bit, that what we know is that the cornerstone and the hallmarks of any successful experimentation program is conducting discipline, reliable, thoughtful, trustworthy, AB test, that will always be the cornerstone of any high performing experimentation team. So do you think it's a case that teams are maybe getting a bit ahead of themselves in some instances and trying to run before they can crawl or walk?
Ken Kutyn 41:38
Yes, maybe a little bit, and I think maybe the software vendors are partially to blame here, because it's an exciting feature and it's sometimes at points in times and more of a competitive differentiator than others. So we love to push stuff like this. I guess I would say to two testing teams’ credit. Yes, it comes up in evaluation criteria all the time, and RFPs, but if you pull the numbers on actual usage, it is quite low. So I think people say, hey, let's ask for this. Why would we not want to have it as an option? And then from some industry numbers, I've heard, it's usually less than 1% or 2% of actual tests are MABs. So I think teams, once they have it, they realize, okay the applications are a little more limited, and we'll try and employ it responsibly.
Gavin Bryant 42:29
Yes that's an interesting stat. Thank you. Thank you so much for sharing that. It's interesting, especially on news sites, where there's obviously a very strong use case for bandits that you check a feature article in the morning, and the header is one version of copy, and then in the afternoon, that it's different. So, yes, they've definitely been running bandits, and particularly optimized for the best winning copy.
Ken Kutyn 43:01
Yes, absolutely great use case and sites like that in media and journalism, often underserved by AB testing, I'd say, because the product team has tools they can test in code and features they ship. But the content teams, the editorial teams, don't always have access to the same tech. And yes, they would definitely benefit from something like an MAB where everything they deliver is so up to the minute, and it's relevant for a day or two only, and they've got a quite a short window to get the most out of it.
Gavin Bryant 43:31
Yes, I think The New York Times maybe, and don't quote me on that exactly, they wrote an interesting article on that a while ago. I'll, I'll see if I can dig it up and post it to the show notes. So just before we wrap up with our closing fastball questions. Ken! so thinking about AI and experimentation. What role will humans have in experimentation going forward?
Ken Kutyn 43:57
Yes, it's a great one, because AI does pose potential to turn a lot of things on their heads. And I wrote a blog last year about whether AI and human led experimentation were kind of at odds, because we see a lot of promises from various software tools like CMSs and experimentation products and personalization products like deliver one to one to everyone, the best experience for the best user, the best time, all this kind of stuff, all powered by AI, right? And I think the question is, if we go down to that one to one level, do we lose the ability to inspect whether it's working? That's the main question for me. And there are ways to preserve measurability and spectability to keep things as an AB test. So for example, if we want to have some personalized content on a landing page based on what we know about the user's past experiences. If we just roll that out to everyone blindly, then we don't really know if it's working. But if we roll that out to half of our users as an experiment, and the other half keep getting the static homepage, now we can deliver one to one to half the people, 50% of the time the other half get something static. So then we can start to have a rigorous AB test on whether algorithms working. We can make tweaks to our AI algorithms, and try and improve them in a measurable way over time. So I think there's some cool applications like that for AI and experimentation. I think in the coming years we're going to see some great help with ideation phases, where we'd be able to show our landing pages, feed some session replay data into some kind of machine learning model, and get some great ideas out of it for what to test. And then human can still be involved in selecting which of those ideas to publish. We're still going to be involved in building and running the tests. Get some help with the analysis process as well. But yes, it's all about amplifying and making humans smarter and more able to run great experiments, rather than replacing as far as I see it.
Gavin Bryant 46:22
Yes, that's what I'm really interesting to see as well. How can ingest all of that information that the previous experimentation results, the qual, the quant, previous hypothesis and outcomes, and then come up with meaningful suggestions to further amplify a site or a page.
Ken Kutyn 46:44
Yes. And I think people are still justifiably so hesitant to hand over the keys to an AI, right? And the silly example, which I know is a bit simplistic is that if we turn it over the keys to AI to optimize every site for conversion rate, all our sites will be adult entertainment in the near future but it does highlight the need for a lot of guardrails in terms of, are we letting AI write brand new text onto a landing page that's personalized, that's unique to every user, because then how can we ensure that text is appropriate and reliable and doesn't contradict our brand messaging doesn't offer unrealistic discounts, doesn't have something offensive in it? So maybe there will be tools that can have that kind of governance and guardrails in place, or maybe that will always be a sticking point. But from what I've seen so far, people are hesitant to give that much kind of unmonitored ability to make changes to an app, to some kind of AI model.
Gavin Bryant 47:53
Interesting to see what comes over coming years. Hey, let's wrap up with our closing fast four questions. These are just some fun and light hearted questions to finish off with. So number one, what are you learning about that we should know about? And think about two cases here, one on the work front and one on the personal front
Ken Kutyn 48:17
Sure, work wise, I'm seeing more and more teams kind of dipping their toe in the water in terms of warehouse native analytics and experimentation. And I think there's a role for that. But again, we don't want to fall into too much hype on something like warehouse powered experimentation when there can still be some limitations. So I think that's one to watch over the coming year or two and see kind of where the right applications for this and where is it not the best fit? So that's something I've been doing bit of reading about lately.
On the personal side, something I've been doing a bit more of lately is baking bread. I love baking sourdough. And, yes, that's been a lot of fun. That's also challenging, rewarding, and requires a bit of experimentation there too.
Gavin Bryant 49:06
What's the secret to a good sourdough? Is it all in the starter?
Ken Kutyn 49:11
Yes, I guess so it's been interesting. How different it is depending on the climate as well. So when you're in a 18 degree house in London, it's a bit different than 26 degree house with the humidity of Singapore, so you need to adjust for that as well.
Gavin Bryant 49:26
It sounds like Singapore's ripe for home brew as well. Ken.
Ken Kutyn 49:32
Yes, not my area of expertise, but I'll take your word for it.
Gavin Bryant 49:36
Number two, what's been your most challenging personal struggle with experimentation?
Ken Kutyn 49:43
Yes, I guess this ties back to what we said before, just not being afraid to start. And something like the statistics can get very complex quickly. And I've done my research and taken my classes and such to get to a level. Where I feel comfortable learning from interpreting AB tests, but I'm not going to go give a talk conference talk tomorrow about statistics. And I think that everyone whether you're in UX, in product and engineering, you yourself to kind of get to level where you feel like you have the baseline competency and you're not making the obvious mistakes. And that can take some time when there's a lot of sophisticated content out there on that. So, yes, don't be afraid, but do your due diligence, I guess.
Gavin Bryant 50:32
Yes. There’s books that have been written on the topic that are hundreds and hundreds of pages deep. So yes. Number three, what's the biggest misconception people have about experimentation?
Ken Kutyn 50:36
Yes, we'll find the right balance there.
Yes, I think we're getting better, but people still view it for simple use cases they think about, you copy, change button, change background image. And I think people would be surprised how much more is going on, and teams who are just starting would be surprised to realize how much further they can take it when it gets into whether it's something like optimizing your parameters and your ML model, or how your search algorithm returns results to the front end, swapping out your tech stack from one cloud provider to another. All these things can be massive AB tests and can be completely transparent to the end user, of course, but they all offer us more reliable, measurable ways to make changes and ship code at the end of the day. So I think that's still a misconception that the teams have that it's all about layout and UI.
Gavin Bryant 51:49
Final question, what's the one thing our audience should remember from our discussion today?
Ken Kutyn 51:55
Yes, I hope that's been encouraging to kind of go back to the point like better to do some testing to do none. Get started. There's lots of great free resource, content conferences out there where you can get your team off the ground and running with their first test. Reach out for help. It's a really fun, supportive community. And yes, I think you'll find it's a great way to stay close to your customers and ultimately learn from them and build better products at the end of the day. So yes, I hope we haven't scared anyone with anything too sophisticated this one just get started. It's better than doing no testing at all.
Gavin Bryant 52:34
Yes great way to finish guessing is in a strategy. And thank you so much for the chat today, Ken. Really appreciate your time. And for those that are interested, the Asia Pacific Experimentation Summit is on the 28th of November. If you have family, friends, colleagues, give them a shout out for the event. We hope to see as many people along there as possible. Great chatting today. Ken, thank you so much.
Ken Kutyn 53:00
Thanks Gavin, appreciate it. Bye.
“ The key premise of agile is to be much closer to our customers. We need to be gathering feedback from customers as often as possible, integrating these insights into development processes and experimentation. Experimentation is an incredible way to do that at scale, with statistical rigour. But, if we are not connecting our experiments to customer needs, the process is broken. We’re doing something fundamentally wrong. ”
Highlights
A/B testing is like a game of chess >> It's competitive. You're up against other teams trying to take your market share who are A/B testing too. Every organisation should throw all available resources behind experimentation. If we don't treat experimentation like a game of chess, where we're constantly trying new moves and new strategies, and trying to stay ahead of competition, you’re going to get left behind
Every opportunity at a point in time is unique - There’s no hard and fast rules or playbooks. Your website and app is not the same as competitors. Your customers are unique to your business. You need to test all of your assumptions - What is the product and how do we package it? How do we communicate features? What is the price? How do we onboard new customers?
Try to not discourage teams from performing their first experiment - Sure, teams will make mistakes along the way and they may not discover a statsig uplift. However, lots of learning will occur throughout the process. Some experimentation is better than none. Performing an experiment is better than guessing. Try not to scare teams off.
Experimenting in low traffic scenarios - Understand which Stats levers you can pull to still perform experiments on low traffic sites. It’s much easier to conduct experiments where the baseline conversion rate is higher. You need less samples to detect an uplift. 1). Pick the right pages to experiment on 2). Pick the right metrics to measure 3). Determine suitable use cases 4). Make bigger, impactful changes
What does a good experimentation program strategy look like? => To decentralise decision-making you need to centralise governance, tools and technology. Experimentation processes need to be standardised with analytics platforms available to teams to promote autonomy. Metrics and metrics definitions are agreed upon and standardised. Evaluating and analysing experimentation data is standardised to empower product teams to make decisions independently
A solid data and analytics foundation is critical before layering experimentation on top. Baseline data needs to be reliable and trustworthy with discrepancies resolved between teams (I.e., Product & Marketing). When experimentation results are presented to Senior Executives the results need to be trustworthy. You want to avoid people saying “I don’t trust the data”
The first steps for establishing high-velocity experimentation - 1). Elicit behavioural change to develop a data-driven culture 2). Provide psychological safety to make mistakes 3). Openly share learnings, not just wins 4). Ensure that data is accessible, reliable and trustworthy 5). Make experimentation self-service (Get teams whatever they need to perform experiments autonomously, analyse results and launch winners themselves)
Experimentation and Feature Flagging is the lower risk way to launch. It provides teams with a “kill switch” to remove code from Production instantly. Feature Flagging can be a great way to overcome conservative and risk averse leadership teams
Experimentation Blockers - many teams struggle when conducting results analysis, particularly when it comes to inconclusive results. Confidence intervals are often overlooked as a way to understand the difference in performance between the Control and Variant
THE BIGGEST MISTAKE - teams skip the important early stages of crafting a strong hypothesis .. understanding customers, what they’re trying to achieve and their problems. Business problems are frequently conflated as customer problems. Many experimenters are not solving real customer problems that have been identified via thorough Qualitative and Quantitative research
Testing one variant can lead to a Local Maxima. Research suggests that the more variations you test the likelihood of finding a statsig winner increases. Teams often test the most obvious solution that is subject to group think or a Captain’s Call. Try to test more solutions out of left field that are informed by strong insights
The key premise of agile is to be much closer to our customers. We need to be gathering feedback from customers as often as possible, integrating these insights into development processes and experimentation. Experimentation is an incredible way to do that at scale, with statistical rigour. But, if we are not connecting our experiments to customer needs, the process is broken. We’re doing something fundamentally wrong
No business has a single Ideal Customer Profile (ICP) or one user type. All businesses have many different types of customers with unique needs. Segmenting experimentation analysis is critical to identify individual segment/cohort behaviours. Otherwise, you’ll end up optimising your product for a General user that doesn’t exist
Experimentation Document Management - works best when there is an individual in an organisation who takes ownership of the document repository and makes it their passion to standardise the format for all tests
Multi Arm Bandits - only 1% - 2% of total experiments conducted in the Amplitude Experimentation Platform are MAB’s. MAB’s are not a substitute for rigorous, statistically significant A/B tests. Determine suitable use cases to perform MAB’s - best for short-term optimisations where the objective is to maximise conversions (I.e., online news site to test feature article headline copy)
The unanswered question with AI and Experimentation is how many organisations will be willing to hand over the keys to AI? In the short to medium term, humans are still going to have an active role in designing and executing tests. AI could potentially play a role in ideating new solutions, test suggestions or test analysis. The role of AI in experimentation will be making humans smarter to run higher quality experiments, rather than replacing humans
In this episode we discuss:
Why A/B Testing is like a game of chess
Every opportunity is unique at a point in time
Why you shouldn’t discourage teams from experimenting
Experimenting in low traffic scenarios
Using feature flagging as a kill switch to decrease risk
Critical steps for establishing high-velocity experimentation
The biggest mistake experimentation teams can make
Why you should be segmenting experimentation results
To perform Multi Arm Bandits, or not?
How AI will help humans perform higher quality experiments
Common experimentation misconceptions
Why all organisations just need to make a start with experimentation
Success starts now.
Beat The Odds newsletter is jam packed with 100% practical lessons, strategies, and tips from world-leading experts in Experimentation, Innovation and Product Design.
So, join the hundreds of people who Beat The Odds every month with our help.