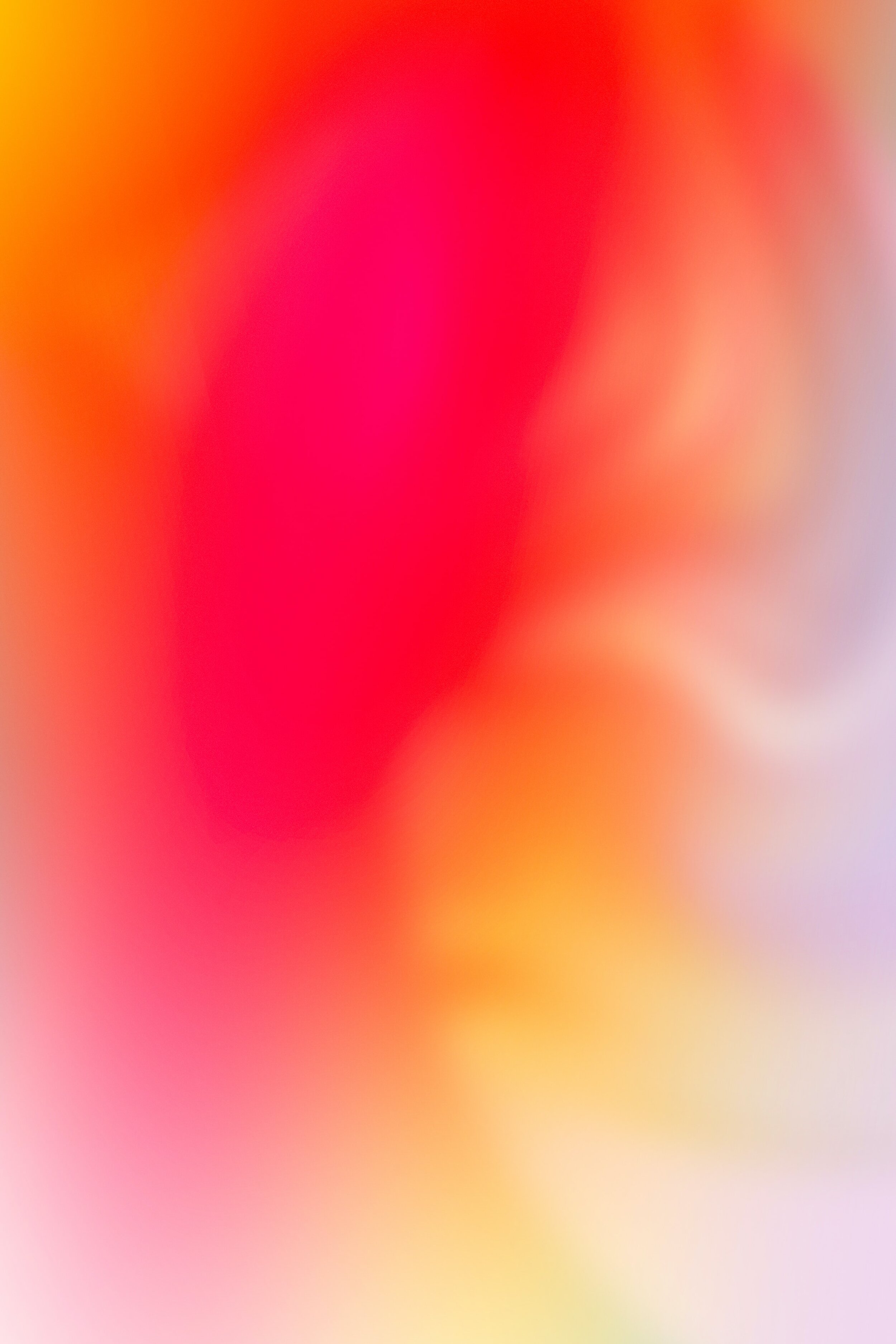
June 16 2022 • Episode 011
Sebastien Polis - Dojo: Experimentation In A Fast-Scaling FinTech
“Experimentation is an opportunity to, at a very early stage, to test assumptions and make sure there is potentially light at the end of the tunnel, and you’re not running completely blind. In big businesses, there’s a lot of survivorship bias. Most product roadmaps will lead nowhere.”
Sebastien Polis is the Experimentation Lead at Dojo. Dojo is a UK based FinTech company that provides payment services to Small and Medium businesses. Dojo enables organisations to take payments through Dojo Go – in-person mobile and portable payments – and Remote Payments with payment links.
Dojo is a sub-brand of Paymentsense, one of Europe’s fastest growing FinTech companies, handling more than $12B worth of sales each year, and processing more than 250M transactions annually. Dojo supplies over 100,000 small businesses in the UK and Ireland, generating revenues over $300M.
Prior to Dojo, Sebastien worked as a Senior Analyst at Healthily, a health tech startup focused on providing triage advice to customers. He was also Data Scientist at DAZN a sports streaming service that provides fans access to live events around the world on demand.
Before jumping into tech, Sebastien worked as a Project Manager at Turner & Townsend using data to provide more accurate costings for major infrastructure projects around the world.
Get the transcript
Episode 011 - Sebastien Polis - Dojo: Experimentation in a Fast-Scaling FinTech
Gavin Bryant 00:03
Hello and welcome to the Experimentation Masters Podcast. Today I would like to welcome Sebastien Polis to the show. Sebastian is currently Experimentation lead at Dojo, a leading UK Fin Tech company that services small to medium enterprise. Dojo is one of Europe's fastest growing Fin Tech companies handling hundreds of millions of transactions per month. Dojo services more than 60,000 customers.
Prior to Dojo, Sebastian worked as a Senior Analyst at healthily, Health Tech startup. He was also a Data Science [phonetic] a Sports Streaming Service that provides access to fans, to events around the world live and on demand. In this episode, we're going to discuss a day in the life of Experimentation at Dojo. Welcome to the show, Sebastian.
Sebastien Polis 01:02
Thank you for having me here.
Gavin 01:03
Ok, let's get started Sebastian. So, I'll ask you for a quick rundown on your background. What's Sebastian's back-story?
Sebastien 01:15
Yes, so that's a pretty interesting story. So, I originally studied Project Management at UCO. I actually started my career in construction, working as a consultant in big projects. And that's where I got my first taste of data. I got involved in a bunch of projects that look to give better cost estimates for our clients on big major Infrastructure Projects.
And that really involved trying to understand the cost elements of big projects, and using data, and data techniques as physical techniques to give our clients a lot better and accurate cost estimates on their projects. And that developed into me, getting more involved in statistics and data.
And I eventually, ended up getting a job at the DAZN as a Data Scientist, where I really explored the area of analytics, engineering, product analytics, and that's where I really got my first touch. With Experimentation, I was working at the DAZN, which is a Sports streaming company, doing loads of front-end analytics, product analytics, understanding subscriptions, what makes customers tick? What is there AHa moment?
And that's where I started working with the Experimentation function, in trying to understand, how to design experiments and how to get some causality on the changes that we were making on the platform? And that was very successful, and I got really interested. And then I eventually, looked for another job at a Health Tech startup, I wanted to get something a little bit smaller.
And I found Healthily, which was a small health startup. And that was really getting started in terms of analytics. And I joined it as a Senior Data Analyst, with the mission to really bring Experimentation into the way that we're developing the product. And that was a very interesting experience for me because it was a very new product. It was very new in the way they were developing things in terms of data. And there was really a chance for me to start in there early and put in the groundwork of Experimentation and overall product analytics.
So, that was a really fascinating experience for me to understand how you set up Experimentation and a process, and framework within a small startup. And we were relatively successful in running a few experiments there and getting to a pretty solid cadence of running one or two experiments a month, which was quite a lot for a small startup.
And they weren’t really used to developing multiple products at the same time. And then after a while, I decided, I wanted to go back to the challenge of doing this, for a much bigger company, which is what Dojo is. And I jumped in Dojo about 10 months ago, with the same mission of kick starting an Experimentation function within the business. So yes, that's been the story so far.
Gavin 04:17
So thinking back to your time in gaming, you mentioned you were intent on, trying to understand, what makes customers tick? So, what was the answer to that question? What did you find that made customers tick?
Sebastien 04:34
Yes, so that's a great question. I think it's an answer that we--- it's a question that we didn't get a full answer to. It's a question that has a lot of answers, I think. We found that the thing, that made customers tick was at least at that time, the ease of access to their favorite sport or their favorite team for their favorite event, right.
And if we could get customers to get into the habit of checking the platform, to find more content about a particular team, we would eventually, get them hooked on the idea of getting subscribed to the DAZN, right. And it was a whole different concept to what you would imagine, you would get a Netflix or, Spotify or, any other subscription-based service, because we deal mostly on live events, right.
And so, we had to come up with pretty clever strategies, I would say on to, how to get those customers to engage and to try, and find when there was no content related to their particular team or, their particular sports, something that would hook them up, right? And there was a lot of clever work done experimenting around recommendations done to customers.
If their particular team was not playing, or their favorite sport, is there something that we can provide them, and then get them into the habit of doing this thing, which at a time was twice a week, every week, right. That's the cadence we wanted customers to come in. And we were relatively successful with that.
We got relatively good uplift and metrics about customers retaining for months, two months, three months, so on, but it was also, it wasn't the golden solution. If you want, there was, obviously, some customers that were falling through the cracks and I left before we could fully answer that question. I don't really think you can truly answer that question completely.
It's something that you have to continuously evaluate and understand, and check what you call model drift, right? You have some answers. And periodically, you would probably want to do an experiment say, are these answers still valid? Is there something else that we should be looking at or, has the market shifted in some way? So yes, that's a quite an interesting problem to tackle at the time.
Gavin 06:51
So, thinking about the Experimentation Program at Healthily, a small startup, what did that Experimentation environment look like?
Sebastien 07:01
So, it was very Ad Hoc in many ways. So, Healthily had about two to three small product teams, of about three or, four people involved being designers, product owners, and so on, and so forth. So, it wasn't a very big scale business. So, we really had to pick the ideas we were having big bets on, right. And it wasn't like, we have a lot of bigger businesses where you have like a very big backlog of ideas you want to test.
We had to be very strategic about the big unknowns, big assumptions that we wanted to look into, because we didn't really have huge sample sizes to play with. And because it was a nascent startup, so, there was a lot of questions about statistical power and achieving some significant results. And the ability to run multiple experiments at the same time, and take into account all of those confounding factors was limited.
And we would have a monthly check in, with the PMs and try, and plan ahead, what experiments we wanted to run at a particular time? And consider trying to do some prioritization around, what was the highest impact or, the highest learning potential? And we didn't really have a very formal prioritization framework, because there was no rush to do it.
We certainly, what we did tend to do was share an idea and come up with an idea about a potential learning, and then share that across the business, and have a lot of input from different people say, oh, do you think this will help us? Do you think is this a valuable learning that we could extract? Is there something else that we should be looking at? And with that prioritization, we will eventually, just launch an experiment, design it, launch it, and we would run it for a month and a half, most of the time to achieve some meaningful statistical certainty.
And then we would try and circulate the learning’s, and just take it on from there, right. So, it wasn't as streamline factory Experimentation, as you want to call it with big backlogs. It was very much an art design process where we're actually looking at, what is the best chance of success in terms of a learning outcome or a significant uplift in some key metrics.
Gavin 09:24
There are very different focuses I imagine looking for more directionality and some signaling whilst trying to, also ensure that the data is as reliable and trustworthy as possible. So, thinking about that experience, I imagine it's quite different to Dojo. How do the two Experimentation environments differ?
Sebastien 09:48
Yes, that's a great question. So, I think there's a little bit of context here in that Dojo is a much larger organization than Healthily was. It is still very early on in its phase to transition, to a product-based organization. So Dojo, who that used to be called Paymentsense for a very long time was promoted early a sales driven organization.
And usually in those types of organizations, there is a space for Experimentation. But in most cases, there isn't really a lot of Experimentation, more on this gut feel and what's worked before. And only recently, over the last year and a half, digital payments has transitioned to Dojo, and in the same process has transitioned to be more of a product led organization.
And it's also grown quite a lot in that timeframe. I think it's expanded ten fold in terms of staff and sales, and everything. So, there's been a very explosive growth. And there's a little bit of a teething pain in that sense of actually trying to understand, what are new processes? How do we go about it?
So, with Healthily, I had a relatively big buy-in from the PMs and it was very easy to do that management of, what should we learn and what should we be striving for. At Dojo, it Is very different, Dojo’s a lot bigger? It has a lot more history and background. There's a lot more stakeholders to manage. So that environment is very different.
And my daily role more than running experiments every day, and designing them every day, which is what I love to do is really going, and meeting stakeholders from different parts of the business to continuously evangelize, and talk, and show the praises of it. And explain that they're doing themselves a disservice by not doing an experiment, right. And they're potentially signing up to doing very long roadmaps that will lead nowhere.
And that Experimentation is an opportunity to, at a very early stage tested assumptions and make sure that there is at least somewhat of a light at the end of the tunnel, and that you're not just going completely blind into it, and hoping for the best, right. And being a relatively big and successful business, it's also a bit of that success by a survivorship bias.
And say, well, we've been doing things like this so far, we are one of the fastest growing Fin Techs in Europe. Clearly, we know what we're doing. And there's a lot of truth to that, right. There's a lot of very smart people who know very well, what they're doing? But there's always room for improvement, there's always room for testing those assumptions and trying to understand is this the best way.
Is this the optimal way to go about certain things? Are we truly solving the customer's problem? Are they doing this? Or are we just thinking doing what we think is best? Because it's worked somewhere else, right. So, those are the main differences I would say is, that one was very simple, easy to convince people and just run an experiment because there was very big unknowns.
And it was a startup and there's loads of new things to conquer. At Dojo, it's a very well established business that is very ambitious and striving for more. And there's a lot more of that stakeholder management, business keys building, if you want to evangelize by, so yes, very different contexts and scenarios.
Gavin 13:13
So, thinking about some of those personal principles or, mental models that you've developed, over your experience, what are some of those key principles you've developed for Experimentation?
Sebastien 13:25
Yes, great question. I think there's, multiple ones. I like tried to get my thoughts here. And I say, the first one is really keep it simple, right. There is always a, when people started getting into Experimentation, there's always a desire to design the most complex, learning riddled experiment. And that will answer all the questions that you have for the next quarter. And it will be this Bible of knowledge.
And sometimes you do get those and you execute them perfectly. And you get such a wealth of knowledge that it's amazing, but they're very rare. And I think that, what you really want to do when you're starting Experimentation is, you want to keep it very simple. And you want to target one particular thing, right. You have one metric; you have one underlying assumption.
That's, the other thing is that when you want to do an experiment, you really want to get to the absolute core of the issue that you're testing on. And I have a great example on this. I was recently in a conversation, where a PM was suggesting a robust solution to a problem that they thought existed.
That required multiple days of multiple Sprints of DEV work to build, and I used the five whys technique. I don't know if you've heard of this. And it's when you question something, why five times. And when we went through that process we eventually, ended up with--- there we didn't really know, if this solution was what our customers want?
We have jumped the gun and said, customers want to have a live representative to talk to, at this point. And there was no actual backing evidence for this, right. There was no, that we hadn't done any services or, questionnaires to our customers about this real need. And I quite like to use this model or, think about this and challenge our PMs and challenge anybody.
We want to say, are you really sure that whatever assumptions that you have is what you're actually testing and what you're trying to solve? Now, you're actually going to learn something that is going to help you with your roadmap, right. So, spend a lot of time thinking about what you're doing and what you want to achieve? And just think about the context and the problem as well, it's very easy to think of, I want to learn this particular, if this is what we need or, we have customers like this.
I want to take out these learning’s. But you also have to take into account the context that you're operating in all the compounding variables that exist within your experiment, and that could potentially impact your results. And if you don't think about that at the early stages, then you might end up with a Post Hoc Analysis, where you have statistical validity, you have results, but your conclusions are not correct, because you have not thought about, what has been happening around it?
And you could be, that will lead them to terrible decision making.
And the last one is test everything, right. There's no reason to think that what worked for business B, which is your competition, is going to work for you? Because if you ask everybody around the business, they'll say, Oh, we're different, right. We're different to our competitors.
On my side, whenever they say that, I'm saying, well, if you're different, why do we assume the same solution is going to work for you? I clearly, maybe does but test it right. Go and test it, and find out if our customers are different to the others, and want the same thing? So yes, test everything, question everything, I guess, and I keep it simple. This will probably be the three key ideas I have.
Gavin 16:57
Let's just circle back to the first point you made and the example that you provide, do you find that's one of the key challenges, that you have in your work is, product teams potentially jumping into solution mode, and not having hypotheses that's really anchored or, grounded in any customer insight.
Sebastien 17:19
Yes, very much so. and I think this is something that you encounter in organizations that again, have grown and been successful doing a particular type of business, and transition to be a more product led approach. Where you need to do a lot of very heavy discovery work to truly understand, what the problem space is? What the customer really wants and to be lean, right?
I think there’s a lot of a problem where executives and stakeholders say, we know what the problem is? We know what we need to build? We know what the next step is? And that's fine. The problem happens when, that we know turns into, you must do [phonetic] and you don't give opportunity for PMs and Designers, and Data Analysts to explore that problem space really, right.
One thing is to say, we know our customers want better customer service and another entire thing. So, therefore we will build a chat bot and say, well, there's quite a few jumps in between. So, there's certainly an issue that I think, it's something that irons itself out when you start building this culture of Experimentation.
And you start moving from the minimum lovable product or, the minimum viable product to what I like to call the minimum viable experiments, where you're doing the absolute bare minimum work to test an underlying assumption, right. And if you can get an experiment out the door in five days, then you get some good results - like learning, you get something out of that and it starts to become addictive.
People start to get hooked into that and say, I want to find this out now. And I want to learn about this. And I want to know if this works? And then once they get into this addiction to knowledge and to learning, and you start to see a transition from product teams to say actually, let's not solutionize. Let's go after those key assumptions. And let's actually go and understand if that really works? If there's, you know, if our current ideas, what is viable, we need to iterate or, if it's just a dead end or, it's detrimental or so on and so forth, right. It's yes. It's something that irons itself out with time and with practice.
Gavin 19:39
Experimentation needs to be experienced to be believed, right. So, just, we're nearly touching on it there. So, what are Dojo’s ambitions with Experimentation?
Sebastien 19:53
I guess that's a great question. And you're asking the exact person for that because it's my mission to experiment, more or less, I lead the program at Dojo. And I say, I've been at Dojo for 10 months with this particular mission. And we're looking to expand the team and really scale up Experimentation, in terms of what our vision/mission for Experimentation - is to turn in Dojo into an evidence based business?
Right, I would love to be in a position, in a year's time, where every single product discussion has an experiment backing it and saying, we've tested this last month. And this is why we're doing X. It has a well crafted pilot and has a lot of evidence at the start, and not just we know, right, from an exec or, this is what customers want.
I would like to be able, to really see very strong data fundamentals whenever you are embarking upon a journey on a roadmap, and that any product also has a very well thought out Experimentation roadmap for the next say, two to three months, where you say, well, we're going to embark onto this, and we're going to try and climb this mountain.
And these are all the multiple paths that we will try. And this is how we're going to go about testing those files. If I can get to that position, and I can do that across the 10s of product teams that Dojo has across multiple different areas, then I would have been very successful in setting up Experimentation culture.
Gavin 21:29
Have you set any specific goals around Experimentation velocity, Experimentation count?
Sebastien 21:35
So, we've not done velocity per se because there's multiple areas in Dojo that have different requirements to be able to run Experimentation properly. And that comes from the nature of being a B2B, B2C business. In terms of an experiment count, yes, there is definitely a goal, more of a North star metric for my team to run a certain number of experiments, per team, per month.
And that's very crafted to each individual team as needs and cadence, and overall product scope. There in the future, as we become a little bit more mature on which of those goals have a number of experiments. I think that's what we will start going into the more refined levels of measuring the success of the program, in terms of velocity, in terms of quality of the experiments, in terms of, as well as spreading the learning’s. And right now, it's--- they're very focused in each individual team and perhaps, some design learning’s that are happening in marketing, are not necessarily passing through to other tribes or, the teams that might benefit out of that, right.
And I think that's something that we obviously, want to address and look to change in the near future. But right now, we're just focusing on trying to get the fundamentals in, and try, and get PMs to think about, oh, I could test that. There's an opportunity here to experiment. And that's the main goal right now.
Gavin 23:09
Yes, really learning how to experiment and to embed those core practices in the team to get the flywheel to start spinning?
Sebastien 23:21
Yes, every good business process needs a flywheel, right. So, if you don't have a flywheel, what are you doing? There is a great one by Aleksander Fabijan that I've used many times. For your own experiments, you get a counter intuitive result, people getting quite interested. You get buy in to get more Experimentation Infrastructure. You run more experiments, and then off you go, right.
And that I, as a team, as an Experimentation Lead, it is my goal to make sure that as a business, we can go faster and faster through that failure, right. And if I get to the point in which I no longer have to say, but let's run an experiment, then great success. And then I can do the really cool part of the job, which is like, let's plan some really clever experiments and let's do multistage things and the state of the art stuff. But at the moment, it's very much me evangelizing, coming in a bit like, a preacher and saying, there's an opportunity here to test something, so let's do it.
Gavin 24:21
So, thinking about the friction in the flywheel at the moment, you just touched on that, which is maybe awareness. Where are the friction points that you see in the flywheel at the moment?
Sebastien 24:36
Yes, no, that's a great question. I think that the main friction point is, you have a very big discrepancy and awareness, and belief in Experimentation or, guess conviction in it, and though just growing a lot in the last year and a half. A lot of people have come on board who come from previous product-based Organizations, are all who have experienced Experimentation are completely bought in, and our allies in my quest to convince the business, to run more experiments.
But at the same time, there's a lot of people who've come in, who come from very commercial backgrounds, from very sales backgrounds, who do not yet have full conviction in this process. And a lot of the friction that comes around Experimentation is that, it is seen as a cost and that the business is not prepared at the moment, right. And there's a bit of, I wouldn't say a failure, but I belief that we know what we must do to reach a base minimum of, where we want to be, and that doing Experimentation will just hinder us in reaching that goal.
And then there are certain key elements that we need to build or, put together as a product. And there's no need to experiment it because it is part of the vision to just have those elements in place, right. And I completely understand that when you have a product vision of what you want to offer, and you get your clients saying, oh, I would love to have XYZ, you want to build that, you want to satisfy those customers, keep them on board and not have them in a churn.
I think that --- there's a lot of work that I can do to reduce the cost of doing an experiment in terms of setting it up, and make having the infrastructure in place that we're doing. And I mean, they're just big enterprise. So, it takes a while to get there. And I think so, some of that friction of seeing it as a cost and seeing the problems of Experimentation, and as extra time or not, or, forgoing some commercial income just to test an idea, which we think is going to work are going to disappear as we start running experiments.
And then as we start presenting those counter intuitive results, then people stop in their tracks for a little bit and think I, now have two options, right. Should I go for the trigger or for Option B? I don't really know. And that's where I want to get to, I don't think like, Experimentation ultimately, is not going to give you the answer. But it's going to give you a set of answers that is going to inform your decision, right.
And that is, it's not the only thing that will make your decision. You will have strategic decisions to put into place or, strategic directives. You'll have design decisions. You'll have multiple things that come and play, rather the market inflation going up, etcetera. Experimentation ultimately, gives you a microscopic view, if you want a detailed view of, what the trade-offs mean? What are you actually sacrificing if you go for A versus you go for B and so on and so forth? And is that worth it? And then you can make a decision based off that
Gavin 27:43
It's a really interesting dichotomy there between being an enabler and blocker, and that perception. But as we're talking about, as the flywheel spins faster, that shifts the narrative back to being an enabler.
Sebastien 28:01
Yes, very much. So, yes, we've already had a few successes where we've presented those counter intuitive results. And I've already started to see some of the executive stakeholders or, senior status stakeholders who've, before were a bit reticent, and now are actually starting to pull Experimentation, right. I've been doing the pushing for about 10 months and trying to find a niche like crook and say, oh, we can test this, let's test an email.
Very simple, very easy to do and as those results have started coming in, I started to see the shift of those senior stakeholders say, oh, you know, I think, I would like to test this. And I would like to get a little bit more evidence about, what we're doing here, right? And it's not the big roadmaps yet.
So yes, it's, you just got to get that flywheel started. And if you manage to get some head turning results at the start, and you should be very strategic about those experiments that you do, and decide to showcase, then you'll slowly start seeing that flywheel will start turning on its own. And it also helps to have really good allies that are keen on Experimentation, right.
But it's certainly getting the ball rolling, and you start seeing people say, hey, I want to do that a little bit of this as well. I want to present on your company-wide meeting and show net positives that we're doing, right. That causality you're saying, we did A, and we caused B that is a very powerful thing. And when you start presenting that people start going, I want a little bit of that, I want to show the successes of my team.
Gavin 29:36
Yeah, it's interesting, the approach that you've taken to start, start small, start local to, as you mentioned, focus on strategic experiments that have business impact or, maybe counter intuitive to the dominant narrative in the business, but through a process of being benefits led and osmosis that's now starting to permeate the business, and to really grow that culture of Experimentation in the business. One of the things that I was really interested in exploring was, and I've read that there's a sense of innovation at Dojo that's real and palpable, what does that feel like on the ground day-to-day?
Sebastian 30:21
Yes, that's very true. Dojo is very innovative. It feels fun. It feels exciting, and sometimes a little bit overwhelming. So, at Dojo, there is probably a new initiative, every two weeks. There is a new buzz word or, a new product that they have not heard of that comes up, which is very exciting when you do Experimentation, because
You might sometimes not be able to do a full A/B test on those new products, because their hardware and it's very expensive to then do 1000s of samples to test the thing. But it gives you the opportunity to just sit down with these guys, and then say, Ok, how are we actually going to validate? What we're trying to achieve? What are we trying to do and get in that really early, right? And it gives me the opportunities, with experimentation to give these products and initiatives, the best chance at success or, at finding the path of least resistance.
Gavin
So, thinking about the Experimentation, team and culture, you mentioned, you've got a small team at the moment. How's the team structured? It sounds like it's a centralized function at the moment?
Sebastian
I really like always the analogy of climbing the mountain, like these new products or, mountains we want to climb. And that's fine. The easiest, fastest path to the peak, right, and we can do that with experiments, with pilots, with different other techniques of causal inference. And I really enjoyed it. And it's fascinating because I get to sit with these super smart people. And then I started challenging their ideas, and they're very receptive to my challenges and proposals of, how we could potentially really get at the core assumption that they are trying to drive or, trying to solve.
Yes, so Dojo is organized in the tribe’s concept, which is familiar to a whole bunch of startups. So, we have, I think its seven to eight tribes at the moment. Each tribe has an embedded data function. So, there's a data organization within the business owners and part of that, and these individuals are all spread amongst the tribes, and they all have their own particular focuses their work with different squads.
And it really works because they get a real sense of, what the squad wants to achieve? What the tribe wants to do? What the data looks like, for that particular area of the business? And they become these embedded specialists. And what the data, the experimentation team does is, we facilitate Experimentation and in each of these individual tribes by relying on those Data Analysts, Data Scientists, right.
So, it is the Data Analysts, Data Scientists responsibility to identify the opportunities for Experimentation with each individual tribe and to actually surface those opportunities, to the experimentation team. And what we try to do is, we try to remove ourselves as blockers by training the Data Analysts, Data Scientists and PMs in the Experimentation techniques, right.
So right now, it's still very nascent. And so they're still, I wouldn't say a blocker, but we're the bottle-neck to try and more of a quality check. We don't really block any experiments at this point, because there's not enough velocity for us to be a hindrance. But what we really want to achieve is disposition, in which the Experimentation team is there to supervise and make sure that everything's running smoothly. But no experiments are going out the door, that makes no sense or, it's going to fail in terms of achieving any earning, right.
It’s not failure in the sense that the initiative will fail. But that experiment is bad in design, and will not give us anything valuable. And we're wasting our time by running that. And ensure that yes, there's a quality to every experiment that goes out the door. And it's been a relatively successful model, and the Experimentation team in itself, the focus is, building tools to help Analysts, to do all of these things. So, for example, we have simulators to help for the power calculations. We are building a set of statistical libraries for the analysis of certain experiments with any statistical test on medians, means etc.
We get involved when there's particularly complex experiments that are coming up or, that have very high business sensitivity or, a lot of stakeholders are looking at that experiment, and we really want to make sure that it is good and well designed, and its objectives are clear. Look for most of the simple easy stuff we just, give it a glance and make sure that yes, there's no showstoppers here, and just let the Data Analyst run the show.
Gavin 35:12
So, thinking about you mentioned, there's some effort around training and capability, and tools. What are some of the other strategies that you've found most effective in trying to really arm the business ready for Experimentation, and to continue building out that culture?
Sebastien 35:31
Yes, great question. So, the number one initiative in that space is the Experimentation Academy, which we've been trialing. So, it sounds ominous or big. But it's simple. It's just a bunch of lectures, talking about the key topics of Experimentation, right. So, about how you design a good hypothesis? How do you make sure it is well backed by data? Do you have any contextual information?
We talk about Power Analysis, and why it's necessary for an experiment to run for a certain amount of time? Why do we, what is the single power? What's the chance of success? We discussed metrics and what metrics are easy to move, and important to move and which ones are very hard to do? So, one metric is very important and Dojo’s, NPS net promoter score that is notoriously difficult to move of an experiment. And it's also relatively subjective. And so it's very hard to deal with.
And when we first started doing Experimentation, a lot of people were like, let's test on NPS and as we had to move away from that, and try and do a lot of work on finding the variables that predict NPS right, and then trying to experiment on those variables and those metrics. So, the Experimentation Academy, we're trying out, we're rolling it out, now, it's been relatively successful. We tried it out with the data or, to level everybody, to be aware of all the experimentation techniques and be able to apply them when necessary.
The other thing that has been really successful and really useful is, putting in a set of standardized templates that guide everybody through the process of designing an experiment, right. And our template is really simple. It starts at the very top with, what is your question? And when do you want to learn? It then asks you to go through, and explain the context of this experiment and any backing evidence. And I'm quite stringent about what people write that?
I want numbers. I want to look at, dashboards. I want charts, I want quotes from customers, I want to have evidence. That this is not just something somebody said, oh, I like this idea. Therefore, we'll do it, right. And then there's a hypothesis actually, a well written crafted hypothesis, we use the format that goes, we know. So, if then--- because it's right and it's very well, if you read that on its own, it gives you pretty much all the context you need, as to, what we're trying to do and what we're trying to achieve, and what problem we're solving? Right
And then we just have that basic information as to, when you expect to run it? What metrics are impacting? Who was involved in this stakeholder or, to stakeholders? And then this is the record of the experiment. So, whenever we share the results, we always send out this fact sheet to everybody. So, there's a very clear record, a transparent record of, what we did? Why we did it? How we went about doing it? And who was involved? So, if you have any further questions, you can always go to these people. And
Gavin 39:02
So, what's your secret for adding spice to the presentation?
Sebastien 39:06
I tried to make it, and particularly, when I'm presenting to the business, when we have these business-wide meetings things we call them. And what I really like to do is I like to be quite a show man.
So, we recently had an experiment where, which we got a counter intuitive result, to what most of the designers thought was going to to the product or, what was going to happen? What our customers thought was going to happen? They all voted for an option.
In the end, it came out to be that another option was better. And when I presented this, I did it in a very show man way. So, I stood in front of the whole business. There was about 200 people in the room, I asked everybody to vote with their hands.
I think it came out that we had a control and two variations. So, it was about at first split everywhere. And I said well, that's great because that means the majority of you are wrong, there's only one winner. That's already a country two.
I showed results one, I've said now, I've shown you door number one, do you want to change your vote? And so that show man approach and trying to make it fun, and trying to make it engaging and you know, have some fun. Most people there hadn't heard of experimentation. But when I got them to vote, when I got them involved, they had a little bit of skin in the game. Although, it was meaningless
If you make something fun, people will start looking forward to it. And if you associate Experimentation with fun, then that will make it easier for the flywheel to start turning. You know, it won't solve all the problems, but you'll definitely get a little bit of a buzz and you certainly one that when you're starting
That has gotten people very excited about Experimentation in many ways. People asked me, oh, when are you going to present another one? They want to hear it and see what we're going to talk about? And that's really interesting and pretty cool, right. So, I think, if you are presenting an experiment, try and make it as fun as an engaging. Try to make it a game show. Try to, maybe your business is very different and very serious, but try and add some humor to it, because it's all about gamification at the end.
We are humans, and humans like to play games. We've been doing it for 1000s and 1000s, of years, and when we have made things fun, things start to roll on their own right. I think there was a few, while now, there is a Volkswagen did this campaign of making things fun, and they have a car on a metro station. And on the stairs, they put like piano keys, and there's loads of viral videos about people playing the keys and take something from that, right.
Gavin 41:39
Seb! I was starting to get excited when you're telling that story. I thought you were going to tell me you're dressed up as a superhero.
Sebastian 41:46
No, well, I might do that. I am certainly not shy enough to, make a little bit of a mockery and make a bit of a joke. Go for it. If that's if you're in a business and you want to project experimentation. And there's certainly that culture of laid back and a bit of fun.
Gavin 42:25
Thinking about your biggest challenges, what have they been so far?
Sebastien 42:29
Biggest challenges, I think we went through some of them. But definitely the educational piece, that's been a challenge. Trying to get my stakeholders to actually understand that Experimentation has a little bit of a cost, but it's actually huge investment in, actually, learning about your product and learning by customers has been a little bit of difficult.
I joined just when Dojo was paymentsense, as was transitioning into Dojo. And we were transitioning into the tribe’s model. And that was a particular challenge because just as I joined, just before this, I was promoting Experimentation into a mature, established business with established ways of working.
And then we said we’re going to change everything. We're going to be product led. We're going to tribes. And suddenly, Experimentation became another priority against multiple competing priorities, some of them which had a lot more stakeholder backing, and then vision, and roadmap right.
And, I had to immediately go into the preacher mode, talk to everybody and anybody who gave me any attention about Experimentation and try, and convince them. And obviously at the start, it was very hard because a lot of people, they had a new team, had a new manager and new stakeholders and had to gel.
And there's this model of team building normally performing or, storming. And I forget the way but it was very much like, storming. And people were discussing, how do we go? What do we do? What are our metrics? And when we came around and said, oh, let's do this cool new thing Experimentation said, we do not have time for that, please. Thank you very much.
So, as that subsided and people started getting into the swing of things, then you started to get a little bit more room to explain Experimentation. Some people get stuck at the start to become a little bit more receptive, right. So, that was a bit of a challenge at the start. The education pieces, and I guess the lack of infrastructure for Experimentation.
There is no unified way to do for example, feature flag, which is the key component and Experimentation, right. The ability to give customers different experiences was not common across the whole company. And I, very quickly had to start a conversation over. We need to acquire a tool? We need to think about, how we're going to implement it? And that also involves a different conversation, of needing engineering resource, engineering staff to be able to work on that project.
So, there was no Experimentation, tooling at Dojo, in different parts of the business. There's multiple products at Dojo. There's the card machine, pay card machines. There's a customer app on iOS and Android. We have other hardware products. We also consider our customer satisfaction, customer service section, a different product that's, there's many different areas that all have different code bases.
At the same time as this re-org and reprioritization going on. So yes, getting the fundamentals in place, and getting the time to convince people. This methodology was certainly hurdles I had to overcome. But we finally managed that we were there. We've gotten a platform, we're starting to use it, we're starting to run experiments, and we're getting good results out of them. And people now, actually, asking to hear more about the wonders of Experimentation, rather than me having to preach to them failing.
Gavin 46:09
Let's just talk quickly, you discussed leadership there a moment ago that you had to really, as you mentioned, get into preacher mode and start to re-influence again, you're competing against projects that had higher priority than Experimentation. How have you found that leadership support in dojo? Could you talk to us about that for a moment?
Sebastien 46:34
Sure, so the leadership who had previous Experimentation experience has been allies and promoters since day one. But obviously, these individuals have other priorities that they need to look at, right. And they've been very helpful at trying to convince other stakeholders, and to help build a case for Experimentation. So, on that side, very grateful to those individuals and the leadership who had come from a more commercial focus background and sales background, weren't necessarily antagonistic blockers or, they just had no understanding of this new methodology or, had barely heard about it.
And in their minds, Experimentation was this thing that you did, when you were a consumer-facing business, with millions of customers AKA, Netflix, Shopify, Spotify or, even Shopify, right. So, Dojo being a b2b business at the time, and will be b2b, b2C. In their minds wasn't really the space for Experimentation. It wasn't a tool that was going to give us loads of benefit. And it was a tool that to them was going to be complicated, to fit into the structure, right.
And ultimately, all of this stems from a lack of understanding of the finer details of Experimentation. So, when you see loads of people present on Ted Talks or, Podcasts about Experimentation, you tend to have the story of the b2C the consumer directly, right. And you've talked about millions of customers, and we get small applets, and so on, and so forth. But there's a whole world of Experimentation, where you can live with Experimentation really.
Where you do techniques, such as synthetic controls, you do things such as you know, well crafted pilots, you look at linear discontinuities, all of these statistical techniques where you can't really run proper AB tests, which are things that we can leverage at Dojo to actually, get a much better understanding of, what causality and what our products are actually doing with our customers, right? And what behaviors we're attracting?
So, we had to commit to either a very large order of these hardware machines, which was quite a very big expense. So, we couldn't really do you know, give it out to 1000 customers and try it out that way. So, we had to be very selective and gave it to only three, I think, restaurants. And we used what is called a synthetic control and a difference in difference technique to measure how the behavior of these customers changed versus another pre selected group that were very similar, right.
There was a lot of education piece around that, and about how we do this? How do we use these techniques? How we can actually shift the mindset? And again, we did one of these quasi Experimentation techniques that helped a lot to educate a particular subset of stakeholders, and we trialed a new payment hardware product with a cost. We have a couple of restaurants around the UK. And the problem we had is that this product was expensive too.
So, think about, imagine you were to give and I'm giving an example a particular product to Starbucks and then you compare that to Costa Coffee, right. And similar cafes, chain cafes, they'll similar have similar clientele. And you can use one to compare to the other. And we got some pretty impressive results out of that experiment as some very counterintuitive results and some second order effects that weren't being expected. And that started to shift that conversation from Experimentation as an expense to, how can we learn out of this opportunity, right?
It wasn't really Experimentation still, there was still this oh, maybe testing is too much for us. But it is more like, what can we do around this space? How can we do this pilot better? And that's the path that we've had with any of the stakeholders and leadership is that, we've had to find a little way in some way, shape or form, do the minimum viable experiment, if you want the minimum viable test. And once that has happened, you start seeing the ice starts to melt a little bit, right. And you get a little bit more space.
And then you know, I go back to the big company wide presentation, when you see another team present, that they change course and actual impact. And there's a verifiable way to demonstrate that very quickly changed the narrative for a lot of people. Because no matter what job you do, you want to be able to demonstrate your own personal impact on what you're doing, right? It doesn't matter what you do, really?
And if somebody comes around and says, I have a technique that will allow you to do that, then you very quickly want to talk to that person, because you know, performance reviews come around. And you say well, look, my team did ABCD. And that's why we moved this metric by 10%. Like, clearly, we're very good. But who doesn't want to be able to do that, right? And so that's the path that we've taken with leadership and the melting has started to happen, like demonstrating the value that not only the business value, but the personal value to yourself, has been really useful or, changing the narrative or, changing the mindsets.
Gavin 52:06
Great example, let's wrap up with the fast four closing now. So, number one, what's frustrating you with Experimentation at the moment?
Sebastien 52:15
That's a great question. Wow, yes, I don't know if frustrated, but I think it's ultimately, is a statistical technique. And there are a lot of business people out there, whenever they see a math formula, will just cower in fear. And I think, there is some work that needs to be done or, can be done. I'd say probably, in making its orientation, more friendly to, I would say, the non-math person in the room in the world, right.
Once you get into it, I mean, maybe I'm biased because it clicks for me, once you start getting into the weeds of Experimentation. And how it works is not that hard. It's not that complicated. Maybe some of the analysis can be complicated, but the basics aren't. So, maybe I need to do some more work on that personally. It is sometimes very frustrating to explain some of those fundamentals. So, predictably Power Analysis run over the idea that you need to have a certain sample size to have a meaningful chance of detecting and effect.
I have used so many examples, and some work and some don't for certain people. I have yet to find that golden ticket, that explains power in the simplest way to everybody and it clicks for everybody. But yes, that's probably my only real frustration is, that it's hard for some people to grasp and grasp the concepts more than just a general idea.
Gavin 53:46
When I spoke to Rohan Katyal, from Facebook (Meta) last week, he made a really good point. And he repeated this numerous times throughout the Podcast, which is abstracting away the complexity of Experimentation should be the core goal to drive uptake.
Sebastian 54:04
Yes, that's very true. If you make it super simple then there's this you remove away a lot of blockers. If you take out the jargon and you explain it in simple layman's terms, people just naturally ignore them too, which is what you want.
Gavin 54:22
Ok, number two, what are you obsessing about right now?
Sebastien 54:24
So, I've touched a little bit on, this is the causal inference techniques. I was familiar with them, but the amount of power to [phonetic] can really leverage for a business like Dojo. It's very interesting and it's something I've deep diving into at the moment. So, I just bought a book called The Causal Inference Mix tape, which looks very promising. I've signed up to a bunch of courses on this. It's certainly tickling that nerd stats geek side of me at the moment. So, yes, it's all of the quasi experimental techniques I would say is, where I'm, my head is at the moment.
Gavin 55:06
Ok, number three. And maybe we just touched on this a little bit. What are you learning about right now that we should know about?
So yes, Causal Inference, if you haven't heard of it, and you're in to Experimentation, go for it. You will be surprised by how many opportunities there is for this, to this technique, and how many new avenues of Experimentation it will open? And areas you imagine are not particularly suited to Experimentation. A perfect example we use in it is, in our customer services section we don't have millions of calls or, hundreds of 1000s of calls in a month. But we need to have a meaningful understanding of what happens over a month, and try, and get some causal impact, right. And that's what we're using these techniques for. So, go for it, go have a look, and certainly will take something out of it.
Gavin 55:57
Final question, resources that you recommend to our listeners to help them on their journey.
Sebastien 56:04
Yes, so the number one resource and I cannot stress this enough, is what, is commonly popularly called The Hippo Book by Ronny Kohavi. This actual title is Trustworthy Online Controlled Experiments, think it's a practical guide to AB testing. If you are in a position where you are working to set up an Experimentation function or, try and learn how all the pitfalls of Experimentation, I would 100% recommend you buy this book, and give it a read because it is absolutely amazing.
There's loads of really useful tips, recommendations and potential problems that you could foresee. I have certainly avoided many issues and many problems by just knowing, what worked, what other people have experimented with and try it out? So, I would recommend it. And then the other big resource I would recommend is, go to all the Major Tech Companies and read about what they're doing in terms of Experimentation.
There is a mountain of information out there that these people are just given out for free. And I am very grateful for them to doing so. And I will be personally adding to that mountain in the near future. But yes, very smart, very clever articles that get you thinking. And the last one is my favorite blog. And I've gotten really great ideas out of it, Towards Data Science in Medium, great examples of different techniques that you can leverage and experiments or, designs that you might not have thought about. So yes, give that one to read, if you're not familiar with it.
Gavin 57:41
Excellent, thank you so much for your time today, Seb. Awesome insight, it’s great to chat.
Sebastien 57:47
Thank you, Gavin. It's been a wonderful chat. Thank you very much.
“When you’re experimenting in a startup, you need to focus on what has the biggest impact and highest learning potential. What are the strategic big bets or big assumptions that you need to investigate? It’s not like a streamlined experimentation factory in a large organisation where you have a big backlog of ideas that you want to test.”
Highlights
Experimentation in startups is different - rather than an experimentation factory of big idea backlogs, it’s about testing the ideas where you want to place big bets. This approach is different to a large organisation, with the focus on being more strategic about testing unknowns and assumptions. You have a limited number of “rolls of the dice” due to lower experiment velocity and customer sample sizes. Use highly targeted experiments to identify areas of strategic success
The role of the Experimentation Lead is not only to design and execute experiments, but to constantly engage with business stakeholders to continuously evangelise the benefits and impact of experimentation
Keep experiments simple - when teams are getting started with experimentation, it’s easy to fall into the trap of designing high-complexity experiments. No one experiment will answer all of your questions. No individual experiment is a bible of knowledge. KISS - target one metric and one key assumption
Practical Tip :- if a business stakeholder has a strongly held belief, drill down into core assumptions using 5 WHY’S. Often there’s no supporting evidence to back up the belief
Think about all the compounding variables in your experiments. If you’re not aware of the upstream and downstream impacts of your experiments it may lead to terrible decision-making
Copying competitors is fraught with danger. You never can know if a competitor solution is working, what is going on in the company and what is driving their success
Minimum Viable Experiments - where you’re doing the absolute bare minimum to test an underlying assumption
Dojo is in the process of transitioning to Product Led Organisation. This is presenting some unique challenges - more time and effort is required for customer discovery to understand customer problem spaces, needs and pain points
Friction points in the Dojo experimentation flywheel 1). Lack of awareness of experimentation 2). Lack of organisational commitment to experimentation 3). Perception of experimentation as a business cost rather than an enabler
Create “PULL” for experimentation by presenting counter-intuitive results to Senior Leaders. Be strategic about which experimentation results you communicate. Try to stop people in their tracks.
Launching a new product is like climbing a mountain. You always want to find the fastest and easiest route to the peak. You can achieve that objective with experiments, well crafted pilots and techniques of causal inference
Dojo Experimentation Academy is a key initiative for training and capability uplift. The experimentation team conduct lectures on key topics - hypothesis formulation, metrics, statistical analysis, how experimentation works etc.
Experimentation Standardisation - Dojo has created a series of templates that guide people through the process of designing good experiments. The templates provide a transparent record of what we did, why we did it, how we went about it and who was involved
MAKE EXPERIMENTATION FUN! - When you’re presenting to business stakeholders create some theatre, buzz and excitement around experimentation. Add humour and create gamification to increase engagement (E.g. a game show format)
In this episode we discuss:
What makes customers tick in online gaming
Experimentation in a small, Health Tech startup
Differences with experimentation in a small, startup vs fast-scaling organisation
Sebastien’s guiding principles for experimentation
Dojo’s experimentation ambitions
Friction points in the Dojo experimentation flywheel
Experimentation team structure
Effective strategies to build experimentation culture and capability
Dojo’s biggest challenges with experimentation
The competing tensions of executive leadership teams
What Sebastien’s learning about right now
Success starts now.
Beat The Odds newsletter is jam packed with 100% practical lessons, strategies, and tips from world-leading experts in Experimentation, Innovation and Product Design.
So, join the hundreds of people who Beat The Odds every month with our help.